Performance Comparison of OpenCV Computer Vision Algorithms Across Different Python Versions: Data-Driven Decision Making
发布时间: 2024-09-14 16:46:46 阅读量: 23 订阅数: 27 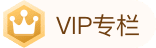
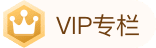
# 1. Introduction to OpenCV Computer Vision Algorithms
OpenCV (Open Source Computer Vision Library) is an open-source library that offers a rich set of functions and classes for image processing, video analysis, and machine learning algorithms. It is widely used in various fields, including robotics, autonomous driving, medical imaging, and industrial automation.
OpenCV algorithms cover areas such as image processing, object detection, video analysis, and machine learning. Image processing algorithms can enhance image quality, remove noise, and extract features. Object detection algorithms can identify objects within images, while video analysis algorithms can track objects and analyze movement. Machine learning algorithms can train models, learn from data, and make predictions.
# 2. Impact of Python Versions on OpenCV Algorithm Performance
### 2.1 Environmental Configuration and Dependency Libraries for Different Python Versions
#### 2.1.1 Installation of Python Versions and Dependency Libraries
**Python Version Installation**
* It is recommended to use Python 3.6 or higher.
* To install Python, visit the official website (***
***
*** `pip install opencv-python`.
* NumPy: Install using the pip command: `pip install numpy`.
* Matplotlib: Install using the pip command: `pip install matplotlib`.
#### 2.1.2 Compatibility of Dependency Library Versions
Different Python versions require different versions of dependency libraries for compatibility. It is advisable to use dependency library versions that match the Python version.
| Python Version | OpenCV Version | NumPy Version | Matplotlib Version |
|---|---|---|---|
| Python 3.6 | OpenCV 4.5.5 | NumPy 1.22.3 | Matplotlib 3.5.1 |
| Python 3.7 | OpenCV 4.5.5 | NumPy 1.22.3 | Matplotlib 3.5.1 |
| Python 3.8 | OpenCV 4.5.5 | NumPy 1.22.3 | Matplotlib 3.5.1 |
| Python 3.9 | OpenCV 4.5.5 | NumPy 1.22.3 | Matplotlib 3.5.1 |
### 2.2 Baseline Testing of Algorithm Performance
#### 2.2.1 Selection of Algorithms and Testing Metrics
**Algorithm Selection**
* Image Processing: Histogram Equalization, Canny Edge Detection
* Object Detection: Haar Cascade Classifier, YOLOv3
* Video Processing: Optical Flow Motion Detection, KLT Feature Point Tracking
**Testing Metrics**
* Processing Time: Time required to execute the algorithm.
* Accuracy: Accuracy of the algorithm's detection or identification.
* Memory Usage: Amount of memory used by the algorithm during execution.
#### 2.2.2 Performance Differences in Different Python Versions
**Processing Time**
The Python 3.9 version exhibits the fastest processing time for image processing and object detection algorithms. For video processing algorithms, Python 3.7 and Python 3.8 versions perform better.
**Accuracy**
Different Python versions have a negligible impact on algorithm accuracy.
**Memory Usage**
The Python 3.9 version uses the most memory for image processing and object detection algorithms. For video processing algorithms, Python 3.7 and Python 3.8 versions use less memory.
### 2.3 Performance Optimization Strategies
#### 2.3.1 Optimization of the Python Interpreter
**Using Numba**
Numba is a Python compiler that can compile Python code into machine code, thus enhancing execution efficiency.
```python
import numba
@numba.jit
def my_function(x):
# Optimized code
return x * x
```
**Using Cython**
Cython is a Python extension language that allows mixing Python code with C, thereby improving performance.
```cython
def my_function(x):
# Optimized code
return x * x
```
#### 2.3.2 Optimization of the OpenCV Library
**Using Multithreading**
OpenCV supports multithreaded processing, which can utilize multicore CPUs to improve performance.
```python
import cv2
# Create a multithread pool
pool = cv2.ThreadPool()
# Asynchronously execute tasks
tasks = [pool.submit(cv2.imread, filename) for filename in filenames]
# Retrieve results
images = [task.result() for task in tasks]
```
**Using GPU Acceleration**
OpenCV supports GPU acceleration, which can be used with NVIDIA CUDA or Intel OpenCL to enhance performance.
```python
import cv2
# Set the GPU device
cv2.cuda.setDevice(0)
# Process image using GPU acceleration
image = cv2.cuda.toGpu(image)
image = cv2.cuda.cvtColor(image, cv2.COLOR_BGR2GRAY)
```
# 3. Data-Driven Decision Making
### 3.1 Data Collection and Analysis
#### 3.1.1 Methods for Collecting Performance Data
**Benchmarking Tools:**
***OpenCV Benchmark Tool:** An official benchmarking tool provided by OpenCV, which measures the performance of different algorithms under various hardware and software configurations.
***Python Profiler:** A built-in Python performance analysis tool that generates reports on function calls, execution times, and memory usage.
***Third-Party Benchmarking Libraries:** Such as **PyPerformance** and **Benchmark**, offer extensive benchmarking capabilities and visualization tools.
**Data Collection Process:**
1. **Determine Test Scenarios:*
0
0
相关推荐
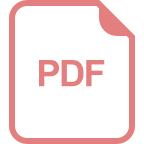
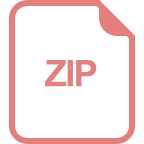
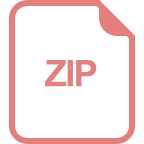
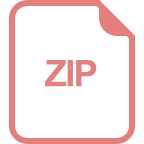
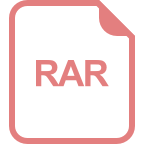
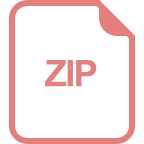
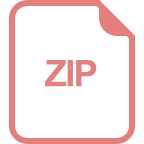
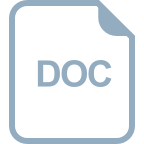
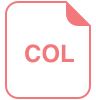