Application of OpenCV and Python Versions in Drone Image Processing: Version Optimization and Performance Enhancement, Improving Aerial Vision
发布时间: 2024-09-14 17:00:25 阅读量: 17 订阅数: 27 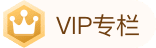
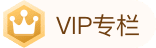
# Application of OpenCV and Python Versions in Drone Image Processing: Version Optimization and Performance Enhancement to Improve Aerial Vision
## 1. Overview of OpenCV and Python in Drone Image Processing
Drone image processing plays a crucial role in modern industrial and commercial applications, offering functions such as real-time environmental perception, target recognition, and obstacle avoidance for drones. OpenCV (Open Source Computer Vision Library) is an open-source computer vision library that offers a wide range of image processing and computer vision algorithms, whereas Python is a high-level programming language renowned for its simplicity and flexibility.
The combination of OpenCV and Python provides a powerful toolkit for drone image processing. OpenCV offers a series of image processing and computer vision algorithms, while Python provides a flexible programming environment that enables developers to easily integrate these algorithms and build custom solutions. This combination allows drones to perform complex image processing tasks, such as target detection, image segmentation, and stitching, thereby enhancing their autonomy and safety.
## 2. Theoretical Foundations of OpenCV and Python Versions in Image Processing
### 2.1 Principles and Algorithms of the OpenCV Image Processing Library
OpenCV (Open Source Computer Vision Library) is an open-source computer vision library that offers a wide range of image processing and computer vision algorithms. These algorithms are based on the fundamental principles and mathematical models of image processing, including:
- **Image Representation:** Images are represented by an array of pixels, with each pixel having a color value (usually RGB or grayscale).
- **Image Transformations:** Image transformation operations are used to modify the appearance or geometry of an image, such as scaling, rotation, translation, and perspective transformation.
- **Image Enhancement:** Image enhancement techniques are used to improve the visual quality of images, such as contrast adjustment, histogram equalization, and sharpening.
- **Image Segmentation:** Image segmentation divides an image into regions with similar features, such as thresholding, region growing, and edge detection.
- **Target Detection and Recognition:** Target detection algorithms are used to locate objects within an image, while target recognition algorithms are used to identify these objects.
### 2.2 Advantages of the Python Programming Language in Image Processing
Python is a high-level programming language that has the following advantages in the field of image processing:
- **Easy to Learn and Use:** Python syntax is simple and clear, making it easy to learn and use, even for beginners.
- **Rich Library Ecosystem:** Python has a rich ecosystem of image processing libraries, such as OpenCV, Pillow, and Scikit-Image, which offer a wide range of functions and algorithms.
- **Scalability and Flexibility:** Python code can be easily integrated with other languages and libraries, making it the ideal choice for building complex image processing applications.
- **Interactive Environment:** Python provides an interactive environment that allows developers to test and debug code quickly, thereby increasing development efficiency.
### Code Example: Converting Images to Grayscale Using OpenCV
```python
import cv2
# Read the image
image = cv2.imread('image.jpg')
# Convert to grayscale image
gray_image = cv2.cvtColor(image, cv2.COLOR_BGR2GRAY)
# Display the grayscale image
cv2.imshow('Gray Image', gray_image)
cv2.waitKey(0)
cv2.destroyAllWindows()
```
**Logical Analysis:**
- The `cv2.imread()` function reads the image and stores it in the `image` variable.
- The `cv2.cvtColor()` function converts the image from the BGR (Blue, Green, Red) color space to the grayscale color space, and the result is stored in the `gray_image` variable.
- The `cv2.imshow()` function displays the grayscale image.
- The `cv2.waitKey(0)` function waits for the user to press any key to close the window.
- The `cv2.destroyAllWindows()` function destroys all opened windows.
### Table: Classification of OpenCV Image Processing Algorithms
| Algorithm Type | Algorithm | Description |
|---|---|---|
| Image Transformations | Scaling, Rotation, Translation | Modify the appearance or geometry of an image |
| Image Enhancement | Contrast Adjustment, Histogram Equalization, Sharpening | Improve the visual quality of an image |
| Image Segmentation | Thresholding, Region Growing, Edge Detection | Divide an image into regions with similar features |
| Target Detection | Haar Cascade Classifiers, HOG Descriptors | Locate objects within an image |
| Target Recognition | SVM, Neural Networks | Identify objects within an image |
# 3.1 Image Preprocessing and Enhancement
### 3.1.1 Image Denoising and Filtering
Image denoising and filtering are important steps in image preprocessing, which can remove noise and interference from images, enhancing the quality of
0
0
相关推荐
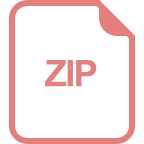
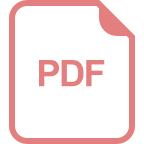
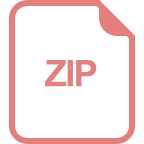
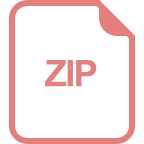
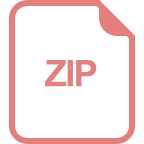
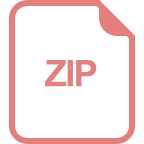
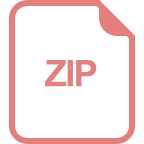
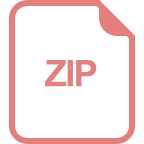
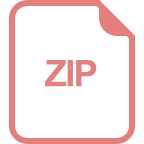