Addressing Data Uncertainty: MATLAB Linear Programming Uncertainty Analysis Modeling and Solution
发布时间: 2024-09-15 09:33:35 阅读量: 18 订阅数: 23 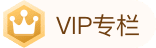
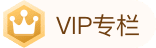
# Dealing with Data Uncertainty: MATLAB Linear Programming for Uncertainty Analysis Modeling and Solving
## 1.1 Basic Concepts of Linear Programming
Linear programming (LP) is a mathematical optimization technique used to maximize or minimize a linear objective function under a set of linear constraints. An LP model consists of the following elements:
- **Decision Variables:** Unknown variables that need to be optimized.
- **Objective Function:** A linear expression to be maximized or minimized.
- **Constraints:** Linear inequalities or equalities that limit the values of decision variables.
The standard form of a linear programming model is as follows:
```
max/min z = c^T x
subject to:
Ax ≤ b
x ≥ 0
```
Where:
- `z` is the value of the objective function.
- `c` is the coefficient vector of the objective function.
- `x` is the decision variable vector.
- `A` is the constraint matrix.
- `b` is the constraint vector.
## 2. Uncertainty Analysis Theory
### 2.1 Types and Sources of Uncertainty
Uncertainty refers to a lack of definite knowledge or the ability to predict future events or outcomes. It can be categorized into the following types:
***Stochastic Uncertainty:** Events or outcomes have a probability distribution and can be modeled using statistical methods.
***Fuzzy Uncertainty:** The scope or boundaries of events or outcomes are unclear and cannot be described by a probability distribution.
***Mixed Uncertainty:** A combination of both stochastic and fuzzy uncertainty.
The sources of uncertainty are diverse, including:
* Errors in data collection and measurement
* Model assumptions and simplifications
* The impact of external factors (such as economic fluctuations, market demand)
### 2.2 Methods of Uncertainty Analysis
There are two main approaches to dealing with uncertainty:
#### 2.2.1 Probabilistic Methods
Probabilistic methods are based on the pro***mon methods include:
***Monte Carlo Simulation:** Estimates the output distribution of a model by randomly sampling and repeatedly calculating.
***Stochastic Programming:** Represents uncertainty parameters as random variables and uses optimization techniques to solve the model.
#### 2.2.2 Fuzzy Mathematical Methods
Fuzzy mathematical methods are based on fuzzy set theory, ***mon methods include:
***α-Cut Method:** Transforms fuzzy sets into a series of deterministic sets and optimizes each set separately.
***Possibility Theory:** Based on the possibility measure of fuzzy sets, evaluates the likelihood of events or outcomes occurring.
**Code Block:**
```
% Probabilistic Method: Monte Carlo Simulation
num_samples = 10000; % Number of samples
samples = randn(num_samples, 1); % Generate normally distributed random samples
mean_sample = mean(samples); % Calculate the sample mean
std_sample = std(samples); % Calculate the sample standard deviation
```
**Logical Analysis:**
This code uses Monte Carlo simulation to estimate the mean and standard deviation of a normally distributed random variable. By generating a large number of random samples, we can approximate the distribution of the random variable and calculate its statistical parameters.
**Parameter Description:**
* `num_samples`: Number of samples, used to generate random samples.
* `samples`: Generated normally distributed random samples.
* `mean_sample`: Sample mean, an approximation of the random variable's mean.
* `std_sample`: Sample standard deviation, an approximation of the random variable's standard deviation.
**Table: Comparison of Uncertainty Analysis Methods**
| Method | Applicability | Pros | Cons |
|---|---|---|---|
| Probabili
0
0
相关推荐
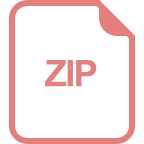
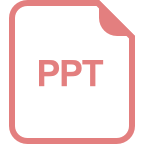
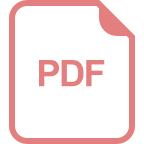





