Efficient Handling of Complex Models: Large-Scale Linear Programming Strategies in MATLAB
发布时间: 2024-09-15 09:28:43 阅读量: 37 订阅数: 31 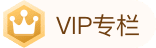
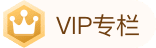
# Efficient Handling of Complex Models: Large-Scale Linear Programming Strategies in MATLAB
## 1. Introduction to Linear Programming
Linear programming is a mathematical optimization technique used to maximize or minimize a linear objective function subject to a set of linear constraints. It is widely applied in fields such as economics, engineering, and management science.
A linear programming model is typically represented as:
```
Maximize/Minimize z = c^T x
Subject to:
Ax ≤ b
x ≥ 0
```
Where:
- z: Objective function
- c: Objective function coefficient vector
- x: Decision variable vector
- A: Constraint matrix
- b: Constraint vector
- x ≥ 0: Non-negativity constraints
## 2. Theoretical Foundations of Linear Programming in MATLAB
### 2.1 Linear Programming Model
**Definition:**
Linear Programming (LP) is a mathematical optimization technique used to maximize or minimize a linear objective function subject to given constraints.
**Standard Form:**
A linear programming model in standard form is as follows:
```
Maximize/Minimize c^T x
Subject to:
Ax ≤ b
x ≥ 0
```
Where:
- `c` is the coefficient vector of the objective function
- `x` is the decision variable vector
- `A` is the constraint matrix
- `b` is the constraint vector
- `<=` denotes inequality constraints
- `>=` denotes equality constraints
### 2.2 Methods for Solving Linear Programming
**Simplex Method:**
The simplex method is an iterative algorithm for solving linear programming problems. It starts with a feasible solution and gradually replaces variables to increase the objective function value until the optimal solution is reached.
**Interior-Point Method:**
The interior-point method is an algorithm based on self-duality for solving linear programming problems. It iterates within the feasible domain, progressively approaching the optimal solution.
**Code Example:**
```matlab
% Define objective function coefficient vector
c = [3; 2];
% Define constraint matrix
A = [1 2; 2 1];
% Define constraint vector
b = [4; 6];
% Solve the linear programming problem
[x, fval, exitflag] = linprog(c, [], [], A, b, [], []);
% Display results
disp('Optimal solution:');
disp(x);
disp('Objective function value:');
disp(fval);
```
**Logical Analysis:**
- The `linprog` function is used to solve linear programming problems.
- The `c` parameter specifies the coefficient vector of the objective function.
- The `A` and `b` parameters specify the constraint matrix and constraint vector.
- The `[]` parameter indicates there are no equality constraints.
- The `x` output variable stores the optimal solution.
- The `fval` output variable stores the objective function value.
- The `exitflag` output variable indicates the solver's exit status.
**Parameter Description:**
| Parameter | Description |
|---|---|
| `c` | Objective function coefficient vector |
| `A` | Constraint matrix |
| `b` | Constraint vector |
| `x` | Optimal solution |
| `fval` | Objective function value |
| `exitflag` | Exit status |
## 3. Practical Applications of Linear Programming in MATLAB
### 3.1 Building a Linear Programming Model
Building a linear programming model is a critical step in solving linear programming problems. In MATLAB, the `linprog` function can be used to build and solve linear programming models. The syntax for the `linprog` function is as follows:
```
[x,fval,exitflag,output] = linprog(f,A,b,Aeq,beq,lb,ub,x0,options)
```
Where:
* `f`: Coefficient vector of the objective function.
* `A`: Coefficient matrix for inequality constraints.
* `b`: Right-hand side vector for inequality constraints.
* `Aeq`: Coefficient matrix for equality constraints.
* `beq`: Right-hand side vector for equality constraints.
* `lb`: Lower bound vector for decision variables.
* `ub`: Upper bound vector for decision variables.
* `x0`: Initial solution vector.
* `options`: Solver options structure.
**Example:**
Consider the following linear programming model:
```
Maximize: z = 3x1 + 2x2
Constraints:
x1 + x2 ≤ 4
2x1 + 3x2 ≤ 12
x1 ≥ 0
x2 ≥ 0
```
Build this model in MATLAB:
```
f = [3, 2];
A = [1, 1; 2, 3];
b = [4; 12];
Aeq = [];
beq = [];
lb = [0, 0];
ub = [];
x0 = [];
[x, fval, exitflag, output] = linprog(f, A, b, Aeq, beq, lb, ub, x0);
```
### 3.2 Solving a Linear Programming Model
After building a linear programming model, the `linprog` function can be used to solve the model. The `linprog` function employs the interior-point method to solve linear programming problems. The interior-point method is an efficient algorithm suitable for large-scale linear programming problems.
**Example:**
Conti
0
0