Accelerating the Solution Process: Effective Means of MATLAB Linear Programming Parallel Computing
发布时间: 2024-09-15 09:29:47 阅读量: 30 订阅数: 31 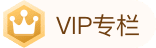
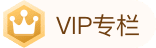
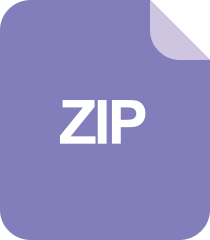
Accelerating MATLAB Performance 源文件:《Accelerating MATLAB Performance》一书的源文件,CRC Press,2014-matlab开发
# Accelerating the Solving Process: Effective Approaches for MATLAB Linear Programming Parallel Computing
## 1. Overview of Linear Programming
Linear programming is a mathematical optimization technique used to solve optimization problems with linear objective functions and linear constraints. It is widely applied in various fields, including resource allocation, production planning, and portfolio management.
The standard form of a linear programming problem is as follows:
```
min c^T x
subject to Ax <= b
x >= 0
```
Where:
* c is the coefficient vector of the objective function
* x is the decision variable vector
* A is the constraint matrix
* b is the constraint vector
The goal of a linear programming problem is to find the values of the decision variables x that minimize the objective function c^T x, while satisfying all constraints.
## 2. Fundamentals of MATLAB Parallel Computing
### 2.1 Concepts and Advantages of Parallel Computing
Parallel computing ***pared to serial computing, parallel computing can significantly improve computational speed and efficiency, especially when dealing with large-scale or complex problems.
The advantages of parallel computing include:
- **Increased Speed:** Parallel computing can divide tasks into smaller subtasks and execute these subtasks simultaneously on multiple processors, thus greatly reducing computational time.
- **Improved Efficiency:** Parallel computing can make full use of computer resources, avoiding the idleness of single-core processors, and improving computational efficiency.
- **Scalability:** Parallel computing can be easily scaled up to use more processors or computers to meet increasing computational demands.
### 2.2 MATLAB Parallel Computing Toolbox
MATLAB provides a powerful parallel computing toolbox, enabling MATLAB users to easily implement parallel computing. This toolbox includes a series of functions and classes for creating and managing parallel pools, task assignment, and synchronization of parallel computing.
#### 2.2.1 Creation and Management of Parallel Pools
A parallel pool is the basic structure used in parallel computing, containing a set of worker processes that execute tasks on different processors. Creating a parallel pool requires the use of the `parpool` function, as shown below:
```matlab
% Create a parallel pool, using all available processors in the system
parpool;
% Create a parallel pool, specifying the number of processors to use
parpool(4);
```
Managing a parallel pool includes starting, stopping, and adjusting the size of the pool. These operations can be performed using the following functions:
- `parpool('open')`: Start a parallel pool
- `parpool('close')`: Close a parallel pool
- `parpool('size')`: Get the number of worker processes in the parallel pool
- `parpool('set', 'NumWorkers', N)`: Adjust the size of the parallel pool, setting the number of worker processes to N
#### 2.2.2 Task Parallelism and Data Parallelism
The MATLAB Parallel Computing Toolbox supports two main parallel computing paradigms: task parallelism and data parallelism.
- **Task Parallelism:** Decompose tasks into smaller subtasks and execute these subtasks simultaneously on different worker processes. Task parallelism is suitable for tasks that are independent of each other.
- **Data Parallelism:** Decompose data into smaller blocks and process these data blocks simultaneously on different worker processes. Data parallelism is suitable for data blocks that are independent of each other.
The MATLAB Parallel Computing Toolbox provides `parfor` and `spmd` functions to implement task parallelism and data parallelism.
- `parfor`: Used for task parallelism, it assigns each iteration of the loop to different worker processes.
- `spmd`: Used for data parallelism, it distributes data blocks to different worker processes and allows communication between worker processes.
## 3. MATLAB Linear Programming Parallel Algorithms
### 3.1 Parallel Simplex Method
#### 3.1.1 Parallel Master-Slave Pattern
The **parallel master-slave pattern** is a classic parallel computing pattern in which a master process is responsible for coordinating task assignment and result collection, while multiple slave processes are responsible for executing computational tasks. In the MATLAB linear programming parallel simplex method, the master process usually maintains the main simplex table, while the slave processes are responsible for computing subproblems and updating the main table.
0
0
相关推荐








