Quick Start Guide to Doris Development: Building Efficient Database Applications
发布时间: 2024-09-14 22:26:26 阅读量: 46 订阅数: 35 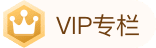
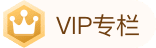
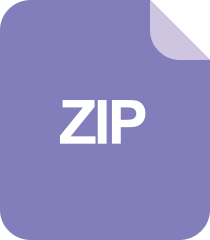
孵化器-doris:Apache Doris(正在孵化)
# 1. Overview of Doris
### 1.1 Introduction to Doris
Doris is an open-source, distributed MPP (Massively Parallel Processing) database designed to handle vast amounts of data and high-concurrency queries. Utilizing a columnar storage engine, it supports high compression ratios and rapid query responses. Doris is widely applied in finance, telecommunications, the Internet of Things, and other fields, offering robust data processing capabilities for real-time analytics, data warehousing, and machine learning scenarios.
### 1.2 Doris Architecture and Features
Doris employs a distributed architecture composed of FE (Frontend) and BE (Backend) components. The FE is responsible for metadata management, query parsing, and optimization, while the BE handles data storage and computation. Doris features include:
- **High Performance:** Columnar storage, parallel computing, and vectorized execution engines enable sub-second query responses.
- **High Availability:** With replication, data sharding, and automatic fault recovery mechanisms, Doris ensures data security and service stability.
- **High Scalability:** Its horizontally scalable architecture supports elastic scaling to accommodate growing data volumes and concurrency demands.
- **Low Cost:** Being open-source with an active community, Doris eliminates the need for costly commercial licenses, reducing enterprise operational expenses.
# 2. Doris Data Modeling
### 2.1 Data Types and Table Design
Doris supports a rich set of built-in data types, including Boolean, integer, floating-point, string, and date-time types. Selecting appropriate data types during table design is crucial for ensuring data accuracy and optimizing storage and query performance.
**Principles for Choosing Data Types:**
***Boolean:** Used for representing true/false values.
***Integer:** For representing integer values, including both unsigned (UNSIGNED) and signed (SIGNED) integers.
***Floating-Point:** For representing floating-point values, including single (FLOAT) and double (DOUBLE) precision.
***String:** For representing textual data, encompassing fixed-length (CHAR) and variable-length (VARCHAR) strings.
***Date-Time:** For representing date and time information, including date (DATE), time (TIME), and datetime (DATETIME).
**Best Practices for Table Design:**
***Select Suitable Primary Keys:** The primary key uniquely identifies a table. Choose columns with high uniqueness and infrequent changes.
***Normalize Data:** Decompose data into multiple tables to avoid redundancy and ensure data consistency.
***Use Foreign Key Constraints:** Define relationships between tables to maintain data integrity.
***Optimize Data Distribution:** Through partitioning and replication strategies, uniformly distribute data across different nodes to enhance query performance.
### 2.2 Partitioning and Replication Strategies
Partitioning and replication are critical data management mechanisms in Doris, and proper partitioning and replication strategies can optimize data storage and query performance.
**Partitioning:**
* Data within a table is divided into multiple partitions based on specific rules, each a separate data block.
* Partitions can be divided based on time, range, or hash values.
* Advantages of partitioning:
* Reduces data scanning scope, improving query performance.
* Simplifies data management, such as data deletion, import, and export.
**Replication:**
* Multiple replicas are created for each partition, stored on different nodes.
* Benefits of replication:
* Enhances data reliability, preventing data loss due to single points of failure.
* Enables load balancing, improving query concurrency.
**Selecting Partitioning and Replication Strategies:**
***Partitioning Strategy:** Choose an appropriate partitioning strategy based on data distribution and query patterns.
***Replication Strategy:** Select the number of replicas based on data importance and reliability requirements.
### 2.3 Data Loading and Management
Doris offers various data loading methods, including import tools, streaming loads, and external tables.
**Import Tools:**
***Doris Loader:** Official command-line tool provided by Doris supports loading data from local files, HDFS, Hive, and other data sources.
***Third-Party Tools:** Tools like Sqoop, DataX, etc., support loading data from relational and NoSQL databases.
**Streaming Loads:**
***Kafka Connector:** Stream data from Kafka into Doris using the Kafka Connector.
***Flink Connector:** Stream data from Flink into Doris using the Flink Connector.
**External Tables:**
* Treat external data sources (like Hive tables, HDFS files) as Doris tables for querying without importing data into Doris.
**Data Management Operations:**
***Data Deletion:** Supports deleting data by partition, time range, or condition.
***Data Modification:** Supports update, delete, and insert operations.
***Data Import/Export:** Supports importing or exporting data to local files, HDFS, Hive, and other data sources.
# 3.1 Query Principles and Execution Plans
#### Query Principles
Doris uses an MPP (Massively Parallel Processing) architecture to divide query tasks into multiple subtasks, which are executed in parallel on different nodes. Each node processes a portion of the data, with the results aggregated and returned to the client.
#### Execution Plans
Doris's execution plan is divided into logical and physical plans. The logical plan describes the semantics of the query, while the physical plan details the specific steps of execution.
**Logical Plan**
The logical plan is generated by the parser, converting SQL queries into a series of logical operators like projection, filtering, and aggregation. Logical operators are connected through data flows, forming a logical execution plan.
**Physical Plan**
The physical plan is generated by the optimizer, transforming the logical plan into a series of physical operators like scanning, sorting, and hash joins. Physical operators are connected through data flows, forming a physical execution plan.
The optimizer selects the optimal physical plan based on factors like data distribution, index information, and query cost.
### 3.2 Indexes and Materialized Views
#### Indexes
Doris supports various indexes, including:
- **Primary Key Index:** For quickly locating data corresponding to primary key values.
- **Secondary Index:** For quickly finding data corresponding to non-primary key values.
- **Bitmap Index:** For rapidly filtering data.
Indexes can significantly enhance query performance, especially when queries involve large amounts of data.
#### Materialized Views
Materialized views are precomputed and stored query results. When queries involve complex computations or aggregations, using materialized views can avoid redundant calculations, thereby improving query performance.
### 3.3 Query Optimization Tips
#### Utilize Indexes
Indexes are one of the most effective methods for improving query performance. When designing table structures, consider creating indexes for frequently queried fields.
#### Avoid Full Table Scans
Full table scans examine all data in a table and are inefficient. Use indexes or partition filters to avoid full table scans whenever possible.
#### Use Partitions
Partitions can divide data into smaller chunks, enhancing query performance. Partition tables based on query patterns and data distribution.
#### Use Materialized Views
Materialized views precompute and store query results, improving query performance. Consider creating materialized views for frequently queried complex computations or aggregations.
#### Optimize Query Statements
Optimize query statements to avoid unnecessary computations and data transfers. Use the EXPLAIN command to view the query execution plan and optimize accordingly.
# 4. Doris Application Development
### 4.1 SQL Programming and API Usage
Doris supports standard SQL syntax and provides rich extensions, enabling users to easily query and manage data. Users can interact with Doris using SQL command-line tools or through JDBC/ODBC drivers in programming languages.
**SQL Programming**
Here's an example of using SQL to query a Doris table:
```sql
SELECT * FROM table_name WHERE column_name = 'value';
```
**API Usage**
Doris also offers APIs for programming languages such as Java, Python, C++, allowing users to interact with Doris programmatically. These APIs provide access to all Doris features, including data querying, data loading, and cluster management.
Here's an example of using the Java API to query a Doris table:
```java
import com.baidu.palo.jdbc.PaloDriver;
import java.sql.Connection;
import java.sql.DriverManager;
import java.sql.ResultSet;
import java.sql.SQLException;
import java.sql.Statement;
public class DorisQueryExample {
public static void main(String[] args) throws SQLException {
// Load Doris driver
DriverManager.registerDriver(new PaloDriver());
// Establish connection
Connection conn = DriverManager.getConnection("jdbc:palo://localhost:8030", "root", "password");
// Create Statement
Statement stmt = conn.createStatement();
// Execute query
ResultSet rs = stmt.executeQuery("SELECT * FROM table_name WHERE column_name = 'value'");
// Traverse result set
while (rs.next()) {
System.out.println(rs.getString(1));
}
// Close connection
rs.close();
stmt.close();
conn.close();
}
}
```
### 4.2 Data Integration and Processing
Doris offers a range of functions to easily integrate and process data.
**Data Integration**
Doris supports importing data from various sources, including filesystems, relational databases, and NoSQL databases. Users can use Doris's provided import tools or programmatic APIs to import data into Doris.
**Data Processing**
Doris provides a series of built-in functions and operators for various data processing operations, including filtering, sorting, aggregation, and joining. Users can also leverage Doris's UDF (User-Defined Functions) mechanism to create custom functions.
### 4.3 Doris Integration with Other Systems
Doris can integrate with other systems to provide a more comprehensive data analysis solution.
**Integration with BI Tools**
Doris supports integration with popular BI tools like Tableau, Power BI, and Google Data Studio. Users can create interactive dashboards and reports to visualize and analyze data within Doris.
**Integration with Machine Learning Platforms**
Doris can integrate with machine learning platforms like TensorFlow and PyTorch. Users can use Doris as a data source for training and inferencing machine learning models and leverage machine learning platforms to build and deploy models.
# 5. Doris Operations and Monitoring
**5.1 Cluster Management and Monitoring**
Doris cluster management and monitoring are primarily achieved through the Doris Manager toolset and Prometheus+Grafana.
**Doris Manager**
Doris Manager is a web-based management interface that offers the following functionalities:
- Monitoring of cluster topology and node status
- Slow query analysis
- Resource usage monitoring
- Alert and notification management
**Prometheus+Grafana**
Prometheus is an open-source monitoring and alerting system, and Grafana is a visualization dashboard and graphing tool. The Doris community provides a Prometheus exporter that can export Doris metrics to Prometheus, which are then visualized and monitored through Grafana.
**5.2 Troubleshooting and Performance Optimization**
**Troubleshooting**
***mon troubleshooting steps include:
- Checking the Doris Manager and Prometheus monitoring dashboards
- Reviewing log files (e.g., fe.log, be.log)
- Using Doris diagnostic tools (e.g., doris-diag)
**Performance Optimization**
Doris performance optimization involves the following aspects:
- **Hardware Optimization:** Selecting appropriate hardware configurations, such as CPU, memory, and storage.
- **Query Optimization:** Using indexes, materialized views, and query tuning techniques to optimize query performance.
- **Cluster Configuration Optimization:** Adjusting cluster configuration parameters, such as replica factor, partition strategies, and resource allocation.
- **Data Loading Optimization:** Using batch loading, parallel loading, and data compression techniques to optimize data loading performance.
**5.3 Doris Ecosystem and Community**
Doris boasts an active community and a rich ecosystem, including:
- **Community Forums:** The Doris community forum is a platform for discussing Doris-related issues.
- **Contributor Community:** Doris welcomes community contributors to participate in code development, documentation writing, and testing.
- **Third-Party Tools:** The community has developed various third-party tools, such as Doris Manager, Prometheus exporter, and data migration tools.
0
0
相关推荐
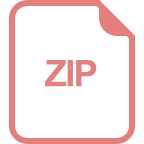
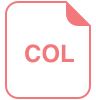
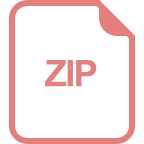
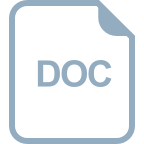
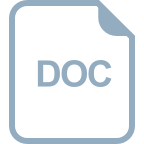
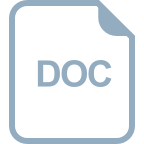
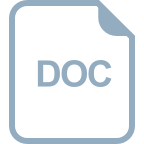