Unveiling Doris Database: The Secret Weapon of the New Generation of Distributed Databases
发布时间: 2024-09-14 22:24:22 阅读量: 25 订阅数: 28 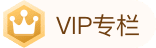
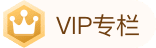
# 1. An Introduction to Doris Database
Doris is an open-source distributed MPP database, designed specifically for big data analytics. It employs columnar storage and vectorized execution engines, enabling efficient processing of massive datasets and rapid query responses. Doris is suitable for a wide range of data analytics scenarios, including data warehousing, real-time analytics, and IoT data processing.
Doris boasts the following features:
- **High Performance:** Columnar storage and vectorized execution engines significantly boost query performance.
- **High Availability:** Utilizing a replica mechanism and failover, Doris ensures data is highly available.
- **Scalability:** With a distributed architecture, Doris can easily scale to meet growing data volume and query demands.
- **Ease of Use:** Doris is compatible with standard SQL and supports various data sources and formats.
# 2. Doris Database Architecture and Principles
### 2.1 Distributed Storage Architecture
Doris adopts a distributed storage architecture, distributing data across multiple nodes for enhanced data processing capabilities and fault tolerance.
#### 2.1.1 Data Sharding and Replicas
To achieve distributed storage, Doris divides data into multiple shards, each stored on different nodes. The shard size can be adjusted based on data volume and query patterns.
In addition, to ensure data reliability, Doris uses a replica mechanism to copy each shard to multiple replica nodes. The number of replicas can be configured based on data importance and availability requirements.
#### 2.1.2 Data Consistency Assurance
In a distributed storage architecture, data consistency assurance is crucial. Doris employs a two-phase commit protocol (2PC) to guarantee data consistency.
The 2PC protocol involves two phases:
1. **Preparation Phase:** The coordinator sends a prepare commit request to all replica nodes; the nodes return a ready state.
2. **Commit Phase:** The coordinator sends a commit request to all replica nodes, which execute the commit operation.
Should a fault occur during the preparation or commit phase, the coordinator will roll back the operation, ensuring data consistency.
### 2.2 Query Engine Optimization
The Doris query engine is highly optimized to support fast and efficient queries.
#### 2.2.1 Columnar Storage and Vectorized Execution
Doris utilizes a columnar storage format, storing data by column rather than by row. This storage format reduces the amount of data read, enhancing query efficiency.
Furthermore, Doris supports vectorized execution, processing multiple data rows at once rather than one at a time. Vectorized execution fully utilizes the parallel processing capabilities of modern CPUs, further increasing query speed.
#### 2.2.2 Materialized Views and Pre-Aggregation
Materialized views are precomputed and stored query results that significantly improve performance for subsequent identical queries.
Doris supports materialized views, allowing users to create them for frequently queried data. When users query this data again, Doris retrieves results directly from materialized views without recalculating, greatly enhancing query efficiency.
Pre-aggregation is another technique to optimize query performance. Pre-aggregation aggregates data onto different dimensions and metrics in advance, reducing the data volume required for queries, thus increasing query speed.
### 2.3 High Availability and Fault Tolerance Mechanisms
Doris offers high availability and fault tolerance to ensure data security and service stability.
#### 2.3.1 Replica Mechanism and Failover
As mentioned, Doris uses a replica mechanism to ensure data reliability. If a replica node fails, Doris automatically replicates data to other replica nodes, ensuring no data loss.
Additionally, Doris supports failover, automatically transferring data and tasks from a failed node to other healthy nodes, ensuring uninterrupted service.
#### 2.3.2 Data Recovery and Disaster Recovery
Doris offers data recovery and disaster recovery mechanisms to handle data loss or disaster scenarios.
Data Recovery: Doris supports data recovery, allowing users to restore lost data from backups.
Disaster Recovery: Doris supports disaster recovery, enabling users to redeploy the Doris cluster on other data centers or cloud platforms and recover data from backups, ensuring business continuity.
# 3. Practical Applications of Doris Database
### 3.1 Data Warehousing and Analysis
#### 3.1.1 Data Modeling and Loading
Data warehouses are enterprise-level data storage systems designed to support decision-making analytics. Doris, as a distributed columnar storage database, has the following advantages in the data warehouse scenario:
- **Columnar Storage:** Doris employs a columnar storage format, grouping similar column data together, greatly enhancing query efficiency.
- **Vectorized Execution:** Doris supports vectorized execution, processing multiple data rows at once, further improving query performance.
- **Materialized Views:** Doris supports materialized views, allowing the precomputation and storage of complex query results, significantly reducing query time.
Doris data modeling follows star or snowflake schemas, where fact tables contain extensive detailed data, and dimension tables hold descriptive information. The data loading process typically involves the following steps:
1. **Data Extraction:** Extract data from source systems, such as relational databases and log files.
2. **Data Transformation:** Convert data into a Doris-compatible format, including data type conversion and data cleansing.
3. **Data Loading:** Use Doris-provided loading tools (such as Stream Load, Broker Load) to load data into Doris tables.
#### 3.1.2 SQL Queries and Analy***
***mon query operations include:
- **Aggregate Queries:** Grouping, aggregating, and sorting data, such as sum, average, maximum, etc.
- **Join Queries:** Connecting relevant data from different tables, such as fact tables and dimension tables.
- **Subqueries:** Embedding other queries within the main query to obtain more complex data.
Doris's query optimizer automatically selects the optimal execution plan based on query conditions and table structures. For instance, for aggregate queries, Doris utilizes materialized views or pre-aggregated tables to accelerate the queries.
### 3.2 Real-time Data Processing
#### 3.2.1 Stream Data Collection and Processing
Doris supports stream data collection and processing, ***mon streaming data sources include:
- **Kafka:** A distributed message queue system suitable for real-time data transfer on a large scale.
- **Flume:** A distributed log collection and processing system suitable for data collection from various sources.
- **Custom Data Sources:** Users can develop custom data source plugins to connect to specific data sources.
Doris provides stream data loading tools (such as Stream Load) that can directly load streaming data into Doris tables. The loading process typically involves the following steps:
1. **Creating Stream Load Tasks:** Specify the data source, Doris table, data format, and loading strategy.
2. **Starting Stream Load Tasks:** Doris continuously reads data from the data source and loads it into the table.
3. **Monitoring Stream Load Tasks:** Check the loading progress, error information, and performance metrics.
#### 3.2.2 Real-time Analysis and Visualization
Doris supports real-time analysis and visualization, ***mon real-time analysis tools include:
- **Doris Dashboard:** An interactive dashboard for creating and managing dashboards that provide real-time data visualization.
- **Third-party BI Tools:** Such as Tableau, Power BI, can connect to Doris to create interactive visualizations.
Doris's real-time analysis capabilities enable enterprises to quickly respond to business changes, promptly identify issues, and take action.
### 3.3 IoT and Edge Computing
#### 3.3.1 Sensor Data Collection and Storage
Doris can be used to store and manage massive sensor data from IoT devices. Sensor data typically has the following characteristics:
- **High Concurrency:** IoT devices continuously generate a large amount of data, requiring high concurrency processing capabilities from the database.
- **Large Data Volume:** Sensor data often includes a significant amount of time-series data, necessitating high storage capacity from the database.
- **Structured Data:** Sensor data usually has a clear data structure, making it suitable for columnar storage formats.
The columnar storage and vectorized execution features of Doris are well-suited for processing IoT sensor data. Additionally, Doris supports time-series data compression, effectively reducing storage costs.
#### 3.3.2 Edge Computing and Data Preprocessing
Edge computing involves data processing near the data source to reduce data transmission latency and costs. Doris can be deployed on edge devices to perform data preprocessing and filtering, then transmit the processed data to the cloud for further analysis.
Edge computing significantly improves the responsiveness and efficiency of IoT applications. Doris's lightweight and scalability make it an ideal choice for edge computing scenarios.
# 4. Advanced Applications of Doris Database
### 4.1 Data Science and Machine Learning
The Doris database has extensive applications in data science and machine learning, offering data scientists and machine learning engineers powerful data processing and analysis capabilities.
#### 4.1.1 Data Preparation and Feature Engineering
Before training machine learning models, data preparation and feature engineering are crucial. Doris provides efficient data loading and transformation functions for rapid processing of massive data and supports user-defined functions and extension modules for complex data transformation and feature engineering tasks.
For example, the following code block demonstrates how to preprocess data and extract features using Doris's built-in functions and user-defined functions:
```sql
-- Load raw data
LOAD DATA INFILE "data.csv" INTO TABLE raw_data;
-- Use built-in functions for data transformation
CREATE TABLE preprocessed_data AS
SELECT
user_id,
CASE
WHEN age < 18 THEN 'Minor'
WHEN age >= 18 AND age < 65 THEN 'Adult'
ELSE 'Senior'
END AS age_group,
gender,
city
FROM raw_data;
-- Use user-defined functions for feature extraction
CREATE FUNCTION get_user_profile(user_id INT) RETURNS STRING;
-- ...User-defined function implementation...
CREATE TABLE user_profiles AS
SELECT
user_id,
get_user_profile(user_id) AS user_profile
FROM preprocessed_data;
```
#### 4.1.2 Machine Learning Model Training and Evaluation
Doris supports integration with popular machine learning frameworks (such as TensorFlow, PyTorch), allowing users to directly train and evaluate machine learning models in the Doris database.
For example, the following code block shows how to use Doris's UDF (User-Defined Function) interface to train a simple linear regression model:
```sql
-- Create UDF
CREATE FUNCTION train_linear_regression(data_table STRING) RETURNS DOUBLE;
-- ...UDF implementation...
-- Train model
SET @model = train_linear_regression('user_profiles');
-- Evaluate model
SELECT
user_id,
predicted_value,
actual_value,
predicted_value - actual_value AS error
FROM user_profiles
JOIN (
SELECT
user_id,
@model(user_profile) AS predicted_value
) AS predictions
ON user_id = user_id;
```
### 4.2 Geographic Spatial Data Processing
Doris provides robust geographic spatial data processing capabilities, supporting the storage, management, querying, and analysis of geographic spatial data.
#### 4.2.1 Storage and Management of Geographic Spatial Data
Doris supports various geographic spatial data types, such as points, lines, polygons, and multi-polygons, and offers efficient geographic spatial indexing for quick retrieval and location of geographic spatial data.
For example, the following code block shows how to load geographic spatial data into the Doris database:
```sql
CREATE TABLE geospatial_data (
id INT,
name STRING,
location GEOGRAPHY
);
LOAD DATA INFILE "geospatial_data.csv" INTO TABLE geospatial_data;
```
#### 4.2.2 Spatial Queries and Analysis
Doris supports a variety of spatial query and analysis functions, such as spatial range queries, nearest neighbor queries, and spatial aggregation queries.
For example, the following code block demonstrates how to use Doris's spatial query functionality to find all geographic spatial objects within a specified area:
```sql
SELECT
*
FROM geospatial_data
WHERE
ST_Contains(ST_GeomFromText('POLYGON((1 1, 10 1, 10 10, 1 10, 1 1))'), location);
```
### 4.3 Custom Functions and Extensions
Doris allows users to write custom functions and extension modules to extend the database's functionality and processing capabilities.
#### 4.3.1 Writing and Using Custom Functions
Custom functions can be used to perform complex data transformations, feature extractions, or other custom operations. Doris supports writing custom functions in various programming languages (such as Java, Python, C++).
For example, the following code block shows how to write a custom function to calculate the distance between two geographic spatial objects:
```java
public class DistanceFunction implements UDF {
@Override
public Object evaluate(Object[] args) {
if (args.length != 2) {
throw new IllegalArgumentException("Invalid number of arguments");
}
Geography location1 = (Geography) args[0];
Geography location2 = (Geography) args[1];
return location1.distance(location2);
}
}
```
#### 4.3.2 Developing and Integrating Extension Modules
Extension modules can be used to extend Doris database functions, such as adding new data sources, storage engines, or analysis algorithms. Doris provides a flexible extension mechanism that allows users to develop and integrate their own extension modules.
For example, the following code block demonstrates how to develop an extension module to read and process CSV files:
```c++
#include "extension_base.h"
class CSVReaderExtension : public ExtensionBase {
public:
CSVReaderExtension() : ExtensionBase("csv_reader") {}
virtual Status init() override {
// ...initialize extension module...
}
virtual Status execute(const std::vector<TExprNode*>& args,
TExprNode** result) override {
// ...execute CSV reading operation...
}
};
```
# 5. Future Development and Prospects for Doris Database**
As an excellent analytical database, Doris will continue to experience rapid growth and expand its application areas in the future. The following is an analysis of the future development and prospects for the Doris database:
### 5.1 Cloud-native and Containerization
With the proliferation of cloud computing, cloud-native technology has become a trend in database development. Doris will further embrace the cloud-native architecture, supporting deployment and management on container orchestration platforms such as Kubernetes. This will streamline the deployment and operations of the Doris database and enhance its elasticity and scalability.
### 5.2 Deep Integration with AI and Machine Learning
AI and machine learning technologies are reshaping industries, and Doris will also deeply integrate with AI technology. By integrating with machine learning algorithms, the Doris database can achieve smarter data analysis and predictions, providing users with deeper insights.
### 5.3 Ecosystem and Community Development
Doris has an active community and ecosystem. In the future, Doris will continue to strengthen cooperation with other open-source projects and communities, building a more comprehensive ecosystem. With the collective efforts of the community, the Doris database will continuously improve its functionality and provide users with a richer range of application scenarios.
0
0
相关推荐
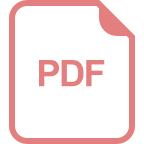
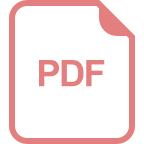
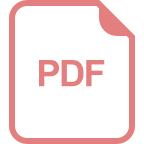
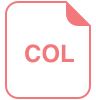
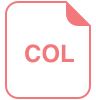
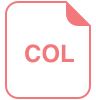
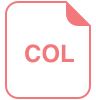
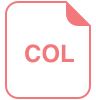
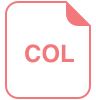
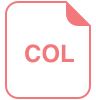