Ensuring Stable Operation of Databases: Best Practices for Doris Database Maintenance
发布时间: 2024-09-14 22:29:30 阅读量: 9 订阅数: 18 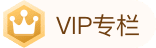
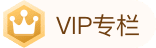
# Ensuring Stable Database Operations: Best Practices for Doris Database Maintenance
## 1. The Basics of Doris Database
Doris database is an MPP (Massively Parallel Processing) based analytics database designed for handling large datasets. Its core advantages include fast queries, high throughput, and low latency.
Doris employs a columnar storage format, storing data by column rather than by row. This method significantly improves query efficiency, especially when dealing with large volumes of data and complex queries. Moreover, Doris supports materialized views, which can pre-calculate and store query results to further enhance querying speed.
## 2. The Theory of Doris Database Operations
### 2.1 Doris Database Architecture and Principles
#### 2.1.1 Doris Database Storage Structure
Doris utilizes a columnar storage structure, storing data by column rather than by row. This structure has the following advantages:
- High data compression rates: Columnar storage effectively compresses data as the same column tends to have similar values, which can be encoded and compressed using a data dictionary.
- Speedy queries: When a query involves specific columns, columnar storage allows for reading only the required columns, rather than the entire row of data, thereby accelerating query speed.
- Excellent scalability: Columnar storage is easy to scale. Adding new columns only requires appending them at the end, without the need to reorganize the entire data table.
The storage structure of Doris primarily consists of the following components:
- Metadata: Contains table structure, partition information, and replica information.
- Data files: Store actual data in a columnar format.
- Index files: Store index information of data files for quick data location.
- Bloom Filter: A probabilistic data structure used for quickly determining whether data exists.
#### 2.1.2 Doris Database Query Engine
The Doris query engine employs an MPP (Massively Parallel Processing) architecture, capable of breaking down query tasks into multiple sub-tasks and executing them in parallel. This architecture boasts the following benefits:
- High throughput: The MPP architecture can process multiple queries simultaneously, enhancing query throughput.
- Low latency: Parallel execution reduces query latency and improves response speed.
- Excellent scalability: The MPP architecture is easy to scale. To improve query performance, simply add more computing nodes.
The Doris query engine primarily consists of the following components:
- Query Coordinator: Responsible for receiving query requests and breaking them down into multiple sub-tasks.
- Compute Nodes: Execute sub-tasks and return results.
- Result Merger: Merges results from compute nodes and returns them to the client.
### 2.2 Doris Database Operation Metrics
#### 2.2.1 System Performance Metrics
System performance metrics reflect the overall operational status of the Doris database system, primarily including the following metrics:
| Metric | Description |
|---|---|
| QPS | Queries per Second |
| TPS | Transactions per Second |
| Latency | Average latency for queries or transactions |
| CPU Usage | CPU utilization |
| Memory Usage | Memory utilization |
| Disk IO | Disk read and write speeds |
#### 2.2.2 Data Quality Metrics
Data quality metrics reflect the accuracy and integrity of data within the Doris database, primarily including the following metrics:
| Metric | Description |
|---|---|
| Data Integrity | Whether data is complete and without loss or damage |
| Data Accuracy | Whether data is accurate without errors or deviations |
| Data Consistency | Whether data is consistent across different replicas |
| Data Timeliness | Whether data is the most up-to-date without delays
0
0
相关推荐
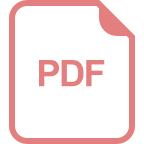
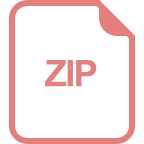
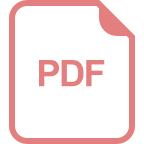





