res = least_squares( 函数使用
时间: 2024-03-05 12:50:25 浏览: 30
least_squares函数是一个用于非线性最小二乘问题求解的函数,它可以通过最小化目标函数来拟合数据。
函数使用方法如下:
```python
from scipy.optimize import least_squares
# 定义目标函数
def fun(x, t, y):
return x[0] * np.exp(-x[1] * t) - y
# 初始参数值
x0 = np.array([1, 1])
# 调用least_squares函数
res = least_squares(fun, x0, args=(t, y))
# 打印拟合结果
print(res.x)
```
其中,fun为定义的目标函数,x0为初始参数值,args为额外的参数传递,res.x为最终拟合得到的参数值。
相关问题
# 求解每个地面点的物方坐标 for i in range(n_points): # 获取该地面点在至少6幅影像上的像点坐标 coord = np.array([points[i]['coord'][j] for j in range(6)]) x = np.array([points[i]['x'][j] for j in range(6)]) y = np.array([points[i]['y'][j] for j in range(6)]) # 使用最小二乘法求解共线方程 param0 = [...] # 初始化参数 res = least_squares(least_squares_eq, param0, args=(coord, x, y, target)) param = res.x中使用最小二乘法求解共线方程的详细代码
共线方程的求解可以使用最小二乘法来实现。最小二乘法的思想是,通过最小化误差的平方和来求解参数的最优解。在这里,误差指的是每个像点坐标与共线方程计算得到的摄站中心坐标和地面点物方坐标之间的距离。
以下是求解共线方程的详细代码:
```python
# 定义共线方程
def collinearity_eq(param, coord, x, y):
# param: 摄站中心坐标和地面点物方坐标
# coord: 影像上的像点坐标
# x, y: 影像像平面坐标系中的坐标系
# 计算像点坐标与摄站中心的距离
dx = coord[:, 0] - param[0]
dy = coord[:, 1] - param[1]
dz = coord[:, 2] - param[2]
# 计算共线方程,返回误差
eq = param[3] * x + param[4] * y + param[5] - dz - param[6] * dx - param[7] * dy
return eq
# 定义最小二乘法求解函数
def least_squares_eq(param, coord, x, y, target):
res = collinearity_eq(param, coord, x, y) - target
return res
# 求解共线方程
param0 = [...] # 初始化参数
res = least_squares(least_squares_eq, param0, args=(coord, x, y, target))
param = res.x
```
其中,`param0`是共线方程的参数的初始值,需要根据实际情况设置。`least_squares`函数可以通过传入求解函数和其他参数来求解最小二乘法的最优解,这里的求解函数是`least_squares_eq`。`args`参数用于传入额外的参数,这里传入了`coord`、`x`、`y`和`target`,分别表示像点坐标、影像像平面坐标系中的坐标、共线方程的目标值和共线方程的参数。函数返回值`res`是一个包含最小二乘法求解结果的对象,其中的`x`属性即为共线方程的参数的最优解。
import numpy as np from scipy.optimize import least_squares import matplotlib.pyplot as plt # 读取.pts文件 # ... # 定义共线方程 def collinearity_eq(param, coord, x, y): # param: 摄站中心坐标和地面点物方坐标 # coord: 影像上的像点坐标 # x, y: 影像像平面坐标系中的坐标系 # 计算像点坐标与摄站中心的距离 dx = coord[:, 0] - param[0] dy = coord[:, 1] - param[1] dz = coord[:, 2] - param[2] # 计算共线方程,返回误差 eq = param[3] * x + param[4] * y + param[5] - dz - param[6] * dx - param[7] * dy return eq # 定义最小二乘法求解函数 def least_squares_eq(param, coord, x, y, target): res = collinearity_eq(param, coord, x, y) - target return res # 求解每个地面点的物方坐标 for i in range(n_points): # 获取该地面点在至少6幅影像上的像点坐标 coord = np.array([points[i]['coord'][j] for j in range(6)]) x = np.array([points[i]['x'][j] for j in range(6)]) y = np.array([points[i]['y'][j] for j in range(6)]) # 使用最小二乘法求解共线方程 param0 = [...] # 初始化参数 res = least_squares(least_squares_eq, param0, args=(coord, x, y, target)) param = res.x # 转换为物方坐标系下的坐标 # ... # 可视化结果 # ...中的读取.pts文件部分详细代码
读取.pts文件的代码可以使用Python自带的`open`函数来实现,然后使用`numpy`库的`loadtxt`函数读取数据。下面是一个可能的实现:
```python
# 读取.pts文件
filename = "data.pts"
with open(filename, 'r') as f:
lines = f.readlines()
# 解析数据
n_points = int(lines[0].strip())
points = []
for i in range(n_points):
point = {'coord': [], 'x': [], 'y': []}
n = int(lines[i*8 + 1].strip())
for j in range(n):
data = lines[i*8 + 2 + j].strip().split()
point['coord'].append([float(data[0]), float(data[1]), float(data[2])])
point['x'].append(float(data[3]))
point['y'].append(float(data[4]))
points.append(point)
```
在上述代码中,我们首先使用`open`函数打开.pts文件,然后读取其中的每一行数据。接下来,我们解析数据,将每个地面点的像点坐标和对应的影像编号存储在一个字典中,然后将所有地面点的字典存储在一个列表中。这样,我们就可以在后续的计算中使用这些数据了。
相关推荐
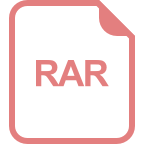
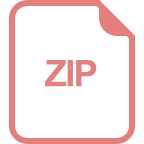












