Unveiling the Culprits Behind MATLAB Crashes: Memory Leakage and Resource Competition, a One-Step Solution
发布时间: 2024-09-13 14:18:30 阅读量: 29 订阅数: 28 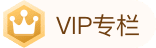
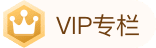
# Unveiling the Culprits Behind MATLAB Crashes: Memory Leaks and Resource Contention, A Single-Stroke Solution
## 1. Investigating the Causes of MATLAB Crashes
MATLAB crashes are a common issue that can be caused by a variety of factors. In this chapter, we will delve into the causes of MATLAB crashes, from memory leaks to resource contention, to help you understand the underlying mechanisms and find effective solutions.
### 1.1 Memory Leaks
Memory leaks are one of the main reasons for MATLAB crashes. When MATLAB fails to release memory that is no longer in use, a memory leak occurs. This results in memory continuously accumulating until it ultimately leads to system crashes.
### 1.2 Resource Contention
Resource contention is another issue that can lead to MATLAB crashes. When multiple threads or processes try to access the same resource simultaneously, resource contention occurs. This can lead to deadlocks, starvation, or race conditions, causing MATLAB to malfunction.
## 2. A Deep Dive into Memory Leaks
### 2.1 Memory Management Mechanisms and Types of Leaks
#### 2.1.1 Memory Allocation and Deallocation in MATLAB
MATLAB employs an automatic memory management mechanism, meaning MATLAB itself is responsible for memory allocation and deallocation. When memory is allocated, MATLAB allocates a block of contiguous memory from a memory pool and returns a pointer to the start address of the memory block. During memory deallocation, MATLAB returns unused memory blocks to the memory pool.
The underlying implementation of memory allocation and deallocation in MATLAB is accomplished through the C language's malloc and free functions. MATLAB encapsulates malloc and free, providing a series of memory management functions such as:
- `zeros`: Allocates and initializes an array to zero
- `ones`: Allocates and initializes an array to one
- `rand`: Allocates and initializes an array to random values
- `free`: Releases memory
#### 2.1.2 Common Causes and Effects of Memory Leaks
A memory leak occurs when MATLAB is unable to release memory blocks that are no longer in use, ***mon causes of memory leaks include:
- **Circular References:** Two or more variables referencing each other, leading to neither being able to be released.
- **Global Variables:** Variables defined outside of functions that MATLAB cannot automatically release.
- **Unreleased Handles:** Handles in MATLAB point to graphical objects, files, or other resources, and unreleased handles can cause memory leaks.
- **Third-Party Libraries:** Third-party libraries may use different memory management mechanisms, leading to memory leaks.
Memory leaks can severely impact MATLAB's performance, including:
- **Memory Shortage:** Memory leaks can lead to MATLAB running out of memory, preventing the allocation of new memory and causing program crashes.
- **Performance Decline:** Memory leaks can slow down MATLAB's operation, as MATLAB needs to spend more time managing memory.
- **Instability:** Memory leaks can cause MATLAB to become unstable, resulting in random crashes or abnormal behavior.
### 2.2 Identifying and Locating Memory Leaks
#### 2.2.1 Memory Analysis Tools and Methods
To identify and locate memory leaks, you can use the following tools and methods:
- **MATLAB Profiler:** MATLAB Profiler can analyze the memory usage of MATLAB programs and identify potential memory leaks.
- **Memory Profiler:** Memory Profiler is a third-party tool for MATLAB that offers more advanced memory analysis capabilities, allowing for a deeper understanding of memory allocation and deallocation.
- **Code Review:** Carefully review the code to find potential memory leaks caused by circular references, global variables, or unreleased handles.
- **Debugging:** Use a debugger to step through the code, observe changes in memory usage, and locate memory leak points.
#### 2.2.2 Debugging and Resolving Typical Leakage Scenarios
**Circular References:**
```matlab
function test()
a = 1;
b = a;
a = [];
end
```
In this example, variables `a` and `b` reference each other, preventing the release of either variable. To resolve this issue, set `a` to `[]` before setting `b` to `[]`.
**Global Variables:**
```matlab
global myVar;
myVar = 1;
```
In this example, `myVar` is a global variable that MATLAB cannot automatically release. To resolve this issue, use `clear global myVar` to release the global variable.
**Unreleased Handles:**
```matlab
figure;
h = gcf;
```
In this example, the variable `h` points to a graphical object handle, and not releasing the handle can cause memory leaks. To resolve this issue, use `close(h)` or `delete(h)` to release the handle.
## 3.1 Threads and Lock Mechanisms
#### 3.1.1 Threads and Parallel Programming in MATLAB
MATLAB supports multithreaded programming, allowing multiple tasks to be executed simultaneously within a single MATLAB process. Threads are lightweight units of execution that share the same memory space and global variables. Parallel programming in MATLAB uses threads to execute code in parallel, thereby increasing computational efficiency.
#### 3.1.2 Types of Locks and Usage Scenarios
Locks are synchronization mechanisms used to control access to shared resources to prevent multiple threads from accessing the same resource simultaneously, leading to inconsistent data or program crashes. MATLAB provides various types of locks:
- **Mutex (Mutual Exclusion Lock):** Ensures that only one thread can access a shared resource at a time.
- **Read-Write Lock (RWLock):** Allows multiple threads to read a shared resource concurrently but permits only one thread to write at a time.
- **Condition Variable:** Used for communication and synchronization between threads.
**Code Block: Creating and Using a Mutex Lock**
```
% Creating a mutex lock
lock = parfeval(@() mutex(), 0);
% Using the mutex lock to protect a shared variable
parfor i = 1:1000
% Acquiring the lock
parfeval(lock, @() lock, 0);
% Accessing the shared variable
sharedVariable = sharedVariable + 1;
% Releasing the lock
parfeval(lock, @() unlock, 0);
end
```
**Logical Analysis:**
This code block creates a mutex lock and uses it to protect the shared variable `sharedVariable` within a parallel loop. The `parfeval` function is used to execute code in a parallel environment, while `lock` and `unlock` functions are used to acquire and release the lock.
### 3.2 Identifying and Resolving Resource Contention
#### 3.2.1 Causes and Dangers of Deadlocks, Starvation, and Race Conditions
**Deadlock:** Occurs when two or more threads wait for each other to release a lock, causing all threads to be unable to proceed.
**Starvation:** Occurs when a thread is unable to acquire a lock for an extended period, preventing it from executing.
**Race Condition:** Occurs when multiple threads access shared resources simultaneously, leading to inconsistent data or program crashes.
#### 3.2.2 Best Practices for Avoiding and Handling Resource Contention
**Avoiding Resource Contention:**
- Carefully design your code to avoid conflicts with shared resources.
- Use appropriate lock mechanisms to control access to shared resources.
**Handling Resource Contention:**
- **Timeout Mechanism:** Set a timeout for locks to prevent deadlocks.
- **Priority Scheduling:** Assign priorities to threads to ensure that important threads acquire locks first.
- **Deadlock Detection and Recovery:** Use tools or techniques to detect and recover from deadlocks.
**Table: Best Practices for Handling Resource Contention in MATLAB**
| Strategy | Description |
|---|---|
| **Mutex Lock** | Ensures that only one thread accesses a shared resource at a time. |
| **Read-Write Lock** | Allows multiple threads to read a shared resource concurrently but permits only one thread to write. |
| **Condition Variable** | Used for communication and synchronization between threads. |
| **Timeout Mechanism** | Sets a timeout for locks to prevent deadlocks. |
| **Priority Scheduling** | Assigns priorities to threads to ensure important threads acquire locks first. |
| **Deadlock Detection and Recovery** | Uses tools or techniques to detect and recover from deadlocks. |
**Code Block: Using a Condition Variable to Avoid Deadlocks**
```
% Creating a condition variable
cv = parfeval(@() condvar(), 0);
% Thread 1
parfeval(cv, @() wait, 0);
% Thread 2
parfeval(cv, @() signal, 0);
```
**Logical Analysis:**
This code block uses a condition variable `cv` to avoid deadlocks. The `wait` function causes Thread 1 to wait for the condition variable to be signaled, while the `signal` function signals Thread 1 to proceed.
## 4. The Single-Stroke Solution
### 4.1 Repair Strategies for Memory Leaks
#### 4.1.1 Optimizing Data Structures and Algorithms
- **Choose the Right Containers:** Avoid containers that are prone to leaks, such as `cell` arrays and `handle` classes. Prefer structured data types like `struct` and `table`.
- **Optimize Algorithms:** Avoid creating unnecessary temporary variables and release variables that are no longer in use promptly. Consider using a memory pool (`memorypool`) to manage large memory allocations.
- **Reduce Closures:** Closures capture external variables, leading to memory leaks. Minimize the use of closures, or use weak references (`weakref`) to prevent leaks.
#### 4.1.2 Employing Memory Management Tools and Techniques
- **MATLAB Profiler:** Use the `profiler` tool to analyze memory usage and identify potential leak points.
- **MAT Files:** Save the MATLAB workspace to a MAT file to release variables that are no longer needed upon reloading.
- **Memory Cleanup Functions:** Write custom functions to periodically clean up memory and release unused objects.
### 4.2 Resolving Resource Contention
#### 4.2.1 Thread Synchronization and Lock Optimization
- **Use Locks:** Use locks in multithreaded environments to protect shared resources and prevent contention.
- **Optimize Lock Granularity:** Use the finest granularity of locks possible to avoid unnecessary blocking.
- **Avoid Deadlocks:** Use deadlock detection tools and follow best practices (such as avoiding nested locks) to prevent deadlocks.
#### 4.2.2 Avoiding Resource Deadlocks and Starvation
- **Priority Scheduling:** Assign higher priorities to critical threads to avoid starvation.
- **Timeout Mechanism:** Set timeouts for lock operations to prevent deadlocks.
- **Resource Pools:** Create resource pools to avoid resource contention.
## 5. Prevention and Best Practices for MATLAB Crashes
MATLAB crashes are a frustrating issue that can lead to data loss and reduced productivity. By understanding the potential causes and implementing best practices, we can effectively prevent and resolve crash issues.
### 5.1 Optimizing Code and Data Structures
**Optimizing Code**
* Avoid memory-intensive operations, such as creating large arrays or loading vast amounts of data.
* Optimize algorithms to reduce memory consumption, such as using sparse matrices or batch processing.
* Avoid recursive function calls, as they may lead to stack overflow.
**Optimizing Data Structures**
* Choose appropriate data structures suited for the data, such as using cell arrays for heterogeneous data.
* Avoid using global variables, as they consume large amounts of memory and may lead to leaks.
* Consider using memory-mapped files to handle large datasets to reduce memory footprint.
### 5.2 Monitoring and Managing Memory Usage
**Monitoring Memory Usage**
* Use MATLAB built-in functions, such as `memory` and `profile`, to monitor memory usage.
* Regularly check Task Manager or Activity Monitor to view MATLAB's memory consumption.
**Managing Memory Usage**
* Use `clear` and `clc` commands to release unused variables and data.
* Avoid creating persistent variables within functions, as they will occupy memory until the function exits.
* Consider using memory management tools, such as `MATLAB Profiler` or `MATLAB Memory Analyzer`, to identify and fix memory leaks.
### 5.3 Avoiding Resource Contention
**Avoiding Deadlocks**
* Use locks to protect shared resources and ensure all threads access them in a consistent manner.
* Avoid nested locks, as they may lead to deadlocks.
**Avoiding Starvation**
* Use fair locks or priority scheduling to ensure all threads have a chance to access resources.
* Avoid busy waiting, as it wastes CPU resources.
### 5.4 Other Best Practices
***Update MATLAB Versions:** Ensure you are using the latest version of MATLAB, as it includes bug fixes and performance improvements.
***Use Code Analysis Tools:** Use MATLAB Code Analyzer or other tools to identify potential memory leaks and resource contention issues.
***Conduct Regular Stress Testing:** Perform stress testing on MATLAB code to identify potential crash issues under high load.
***Use Version Control:** Use version control systems to track code changes and roll back to stable versions.
***Seek Professional Support:** If you cannot resolve crash issues on your own, contact MATLAB technical support or consult experts.
By following these best practices, we can effectively prevent and resolve MATLAB crash issues, ensuring the stability and reliability of our applications.
## 6. Conclusion and Outlook
Reviewing the entire article, we have delved deeply into the causes of MATLAB crashes, the mechanisms of memory leaks, the essence of resource contention, and their respective solutions. Through a comprehensive analysis of these issues, we have gained the following valuable insights:
- MATLAB crashes are often caused by memory leaks, resource contention, or other factors.
- Memory leaks result from MATLAB's inability to release memory blocks that are no longer in use, leading to program crashes.
- Resource contention occurs when multiple threads or processes access shared resources simultaneously, potentially leading to deadlocks, starvation, or race conditions.
- Addressing memory leaks and resource contention requires a comprehensive approach, including optimizing code, using memory management tools, synchronizing threads, and optimizing locks.
- Preventing MATLAB crashes hinges on following best practices, such as using appropriate data structures, optimizing algorithms, avoiding resource deadlocks, and starvation.
Looking to the future, MATLAB's development will continue to focus on enhancing performance, stability, and scalability. As new features and technologies continue to emerge, mastering these solutions is crucial for MATLAB developers. By deeply understanding MATLAB's internal mechanisms and best practices, we can build robust, efficient applications that maximize MATLAB's potential.
0
0
相关推荐
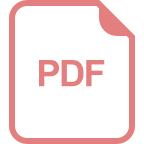
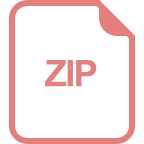
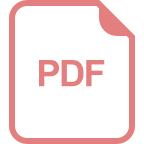
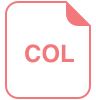
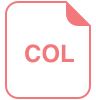
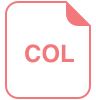
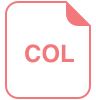
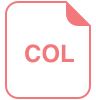
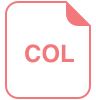