GPU Acceleration Issues in MATLAB Crashes: Exploring and Resolving Graphics Processing Faults to Unleash GPU Potential
发布时间: 2024-09-13 14:30:15 阅读量: 23 订阅数: 25 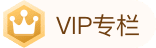
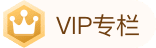
# 1. Overview of GPU Acceleration**
GPU (Graphics Processing Unit) acceleration is a technique that utilizes the parallel computing capabilities of GPUs to speed up MATLAB computations. By assigning computational tasks to the GPU, performance can be significantly improved, especially in applications that involve a large amount of parallel computing.
The principle behind GPU acceleration lies in the GPU's possession of a large number of parallel processing units (CUDA cores), capable of executing multiple computational tasks simultaneously. This is in contrast to the CPU, which typically has only a few cores and can only execute tasks sequentially. Therefore, for applications that require processing vast amounts of data, GPUs can provide a significant performance advantage.
# 2. Theoretical Foundations of GPU Acceleration
### 2.1 Principles of GPU Parallel Computing
A **GPU (Graphics Processing Unit)** is a hardware device specifically designed for processing graphics and video data. Unlike a CPU (Central Processing Unit), a GPU has a massively parallel processing capability, making it well-suited for tasks that require a large amount of parallel computation.
The principle of GPU parallel computing involves breaking down tasks into many smaller subtasks, which are then executed simultaneously on multiple processing cores. This parallel processing approach can significantly increase computing efficiency, especially when dealing with large datasets.
**CUDA (Compute Unified Device Architecture)** is a parallel computing platform developed by NVIDIA, designed for programming GPUs. CUDA allows programmers to write code in the C language and leverage the parallel processing capabilities of GPUs to accelerate computations.
### 2.2 GPU Memory Model and Optimization
GPUs have their own dedicated memory, known as **GPU memory**. Unlike CPU memory, GPU memory has higher bandwidth and lower latency. However, GPU memory is also more limited compared to CPU memory.
The **GPU memory model** consists of several parts:
- **Global Memory:** Shared memory accessible by all threads.
- **Shared Memory:** Shared memory accessible by threads within the same thread block.
- **Local Memory:** Private memory unique to each thread.
Optimizing **GPU memory usage** is crucial for enhancing the performance of GPU acceleration. Here are some optimization tips:
- **Reduce Global Memory Access:** Try to use shared memory or local memory to store data to minimize accesses to global memory.
- **Use Texture Memory:** For tasks such as image processing that require a large amount of texture data, using texture memory can improve performance.
- **Avoid Memory Fragmentation:** Allocate memory wisely to avoid memory fragmentation and improve memory utilization.
**Code Block:**
```c
__global__ void kernel(int *a, int *b, int *c) {
int tid = threadIdx.x;
int blockIdx = blockIdx.x;
int blockDim = blockDim.x;
int gridDim = gridDim.x;
// Calculate each thread's index
int index = blockIdx * blockDim + tid;
// Access global memory
a[index] += b[index];
// Synchronize threads
__syncthreads();
// Access shared memory
c[tid] = a[index];
}
```
**Code Logic Analysis:**
This code block is a CUDA kernel function designed for parallel computation of the sum of two arrays, a and b, and storing the result in array c.
***tid:** Thread ID, representing the current thread's index within the block.
***blockIdx:** Block ID, indicating the current block's index in the grid.
***blockDim:** Block size, representing the number of threads in each block.
***gridDim:** Grid size, representing the number of blocks in the grid.
The kernel function first calculates each thread's index and then uses this index to access the a and b arrays in global memory. It then uses the __syncthreads() function to synchronize threads, ensuring all threads complete their access to global memory before accessing shared memory. Finally, it stores the computed result in the c array located in shared memory.
**Parameter Explanation:**
***a:** Input array 1
***b:** Input array 2
***c:** Output array
# 3.1 Basic MATLAB GPU Programming
**GPU Programming Paradigm**
GPU programming in MATLAB follows the Single Instruction, Multiple Data (SIMD) paradigm, meaning that
0
0
相关推荐
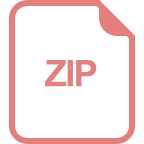
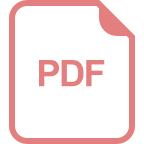
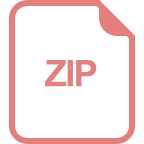
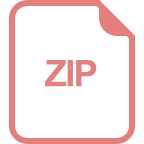
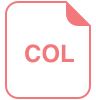
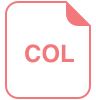
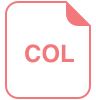
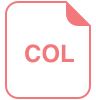
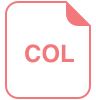