In-Depth Analysis of Insufficient MATLAB Input Parameters: Theory, Practice, and Optimization Strategies
发布时间: 2024-09-14 14:34:30 阅读量: 49 订阅数: 33 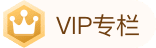
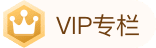
# In-depth Analysis of Insufficient Input Parameters in MATLAB: Theory, Practice, and Optimization Strategies
MATLAB is a high-level programming language widely used for scientific computing and data analysis. In MATLAB, functions and scripts usually require input parameters to perform specific tasks. However, insufficient input parameters can lead to various issues, including uncertainty in function behavior, decreased algorithm performance, and even program crashes.
Insufficient input parameters refer to the situation where the required parameters for a function or script are not provided when executed. This can be caused by various reasons, such as user error, code defects, or issues with external data sources. Understanding the theoretical basis of insufficient input parameters, practical impacts, and optimization strategies is crucial for writing robust and reliable MATLAB code.
# Theoretical Basis of Insufficient Input Parameters
### 2.1 Parameter Types and Missing Mechanisms
Parameters in MATLAB can be classified into the following types:
| Parameter Type | Description |
|---|---|
| Required Parameters | Parameters that must be provided; otherwise, the function cannot execute |
| Optional Parameters | Parameters that can be omitted, using default values if omitted |
| Named Parameters | Parameters specified using name-value pairs |
| Variable-length Parameters | Parameters that can accept any number of arguments |
Parameter missing refers to the situation where required or optional parameters are not provided during a function call. The missing mechanism can be divided into two types:
| Missing Mechanism | Description |
|---|---|
| Explicit Missing | Parameters are explicitly omitted, e.g., `f(x)` |
| Implicit Missing | Parameters use default values, e.g., `f(x, [])` |
### 2.2 Methods for Handling Missing Data
Common methods for handling missing data include:
| Method | Description |
|---|---|
| Ignoring Missing Values | Treat missing values as valid data, which may lead to errors or inaccurate calculations |
| Deleting Missing Values | Remove rows or columns containing missing values, which may lead to data loss |
| Filling Missing Values | Estimate missing values using methods such as mean, median, or interpolation |
The choice of method for handling missing data depends on the specific application and data characteristics. For example, if missing values are randomly distributed, it may be reasonable to ignore them. If missing values are systematic, then deleting or filling them may be more appropriate.
**Code Block 1: Function for Handling Missing Data**
```matlab
function data = handleMissingData(data, method)
% Handles missing data
%
% Input Parameters:
% data - Input data, can be a matrix, vector, or structure
% method - Method for handling missing values, can be 'ignore', 'remove', or 'fill'
%
% Output Parameters:
% data - Processed data
switch method
case 'ignore'
% Ignore missing values
case 'remove'
% Remove rows or columns containing missing values
data = data(~any(isnan(data), 2), :);
case 'fill'
% Fill missing values using the mean
data = fillmissing(data, 'mean');
otherwise
error('Invalid method: %s', method);
end
end
```
**Code Logic Analysis:**
This function processes missing data based on the specified method. It accepts input data and the method of processing as parameters. Depending on the method, it can ignore missing values, remove rows or columns containing missing values, or fill missing values using the mean. If an invalid method is specified, the function will generate an error.
**Parameter Description:**
* `data`: Input data, can be a matrix, vector, or structure.
* `method`: Method for handling missing values, can be 'ignore', 'remove', or 'fill'.
# Practical Impacts of Insufficient Input Parameters
### 3.1 Uncertainty in Function Behavior
When a function's input parameters are insufficient, the behavior of the function may become uncertain. This is because the function does not know how to handle the missing parameters and may produce unexpected results. For example, consider the following MATLAB function:
```
function myFunction(x, y, z)
result = x + y + z;
end
```
If we call this function and only provide two parameters, the function will produce an error:
```
>> myFunction(1, 2)
Error: Not enough input arguments.
```
This is because the function expects three parameters, but we only provided two. To avoid this error, we must provide all three parameters, as shown below:
```
>> myFunction(1, 2, 3)
ans = 6
```
### 3.2 Decreased Algorithm Performance
Insufficient input parameters not only lead to uncertain function behavior but can also cause decreased algorithm performance. This is because the algorithm may not be able to correctly process the missing parameters and may produce inaccurate or inconsistent results. For example, consider the following MATLAB algorithm for calculating the average of a set of numbers:
```
function avg = calculateAverage(numbers)
sum = 0;
for i = 1:length(numbers)
sum = sum + numbers(i);
end
avg = sum / length(numbers);
end
```
If we call this algorithm and only provide one number, the algorithm will produce a NaN (Not a Number) result:
```
>> avg = calculateAverage(1)
avg = NaN
```
This is because the algorithm expects a set of numbers, but we only provided one. To get the correct average, we must provide a set of numbers, as shown below:
```
>> avg = calculateAverage([1, 2, 3, 4, 5])
avg = 3
```
# Optimization Strategies for Insufficient Input Parameters
### 4.1 Parameter Checking and Validation
When writing MATLAB functions, parameter checking and validation are crucial to ensure the validity and completeness of input parameters. This can prevent uncertain function behavior or decreased algorithm performance due to insufficient input parameters.
#### 4.1.1 Manual Parameter Checking
The simplest method for manual parameter checking is to use the `nargin` function, which returns the number of parameters passed to the function. If `nargin` is less than the minimum required number of parameters for the function, an error message or warning can be issued.
```matlab
function myFunction(x, y, z)
if nargin < 3
error('Insufficient input arguments.');
end
% ...
end
```
#### 4.1.2 Automatic Parameter Validation
MATLAB provides an in-built function called `validateattributes`, which allows for automatic validation of input parameters. This function permits the specification of parameter types, sizes, ranges, and other attributes. If any parameters do not meet the specified constraints, an error is thrown.
```matlab
function myFunction(x, y, z)
validateattributes(x, {'numeric'}, {'scalar', 'positive'});
validateattributes(y, {'numeric'}, {'vector', 'numel', 3});
validateattributes(z, {'char'}, {'nonempty'});
% ...
end
```
### 4.2 Default Values and Optional Parameters
In addition to parameter checking and validation, using default values and optional parameters is an effective strategy for handling insufficient input parameters.
#### 4.2.1 Principles for Setting Default Values
When setting default values for parameters, the following principles should be followed:
***Rationality:** Default values should be the most reasonable option in most cases.
***Consistency:** Default values should be consistent with the expected behavior of the function.
***Documentation:** Default values should be explicitly explained in the function documentation.
```matlab
function myFunction(x, y, z)
if nargin < 2
y = 0;
end
if nargin < 3
z = 'default';
end
% ...
end
```
#### 4.2.2 Flexible Handling of Optional Parameters
Optional parameters allow users to specify particular values or use default values when calling a function. This provides greater flexibility and allows users to customize the behavior of the function as needed.
```matlab
function myFunction(x, y, varargin)
% ...
if nargin > 2
z = varargin{1};
else
z = 'default';
end
% ...
end
```
# Real-world Cases of Insufficient Input Parameters in MATLAB
### 5.1 Case Study: Image Processing Function
**Problem Description:**
Consider the following MATLAB image processing function:
```matlab
function [outputImage] = myImageProcessingFunction(inputImage, threshold)
if nargin < 2
threshold = 0.5;
end
% Image processing operations
outputImage = ...;
end
```
This function performs image processing operations and accepts two input parameters: `inputImage` (input image) and `threshold` (threshold). If the `threshold` parameter is not provided, the function uses the default value of 0.5.
**Analysis:**
This function has a problem with insufficient input parameters because if the user does not provide the `threshold` parameter, the function will use the default value. This can lead to unexpected results, especially when the default value is not suitable for a particular image processing task.
### 5.2 Case Study: Machine Learning Model
**Problem Description:**
Consider the following MATLAB machine learning model:
```matlab
function [model] = myMachineLearningModel(trainingData, labels, maxIterations)
if nargin < 3
maxIterations = 1000;
end
% Model training operations
model = ...;
end
```
This function trains a machine learning model and accepts three input parameters: `trainingData` (training data), `labels` (labels), and `maxIterations` (maximum iterations). If the `maxIterations` parameter is not provided, the function uses the default value of 1000.
**Analysis:**
This function also has a problem with insufficient input parameters because if the user does not provide the `maxIterations` parameter, the function will use the default value. This can affect the quality of model training, especially when the default value is not suitable for a specific machine learning task.
### Optimization Strategies
To address the issue of insufficient input parameters in these real-world cases, the following optimization strategies can be adopted:
***Use parameter checking and validation:** At the beginning of the function, use functions like `nargin` and `isnumeric` to check the number and type of input parameters. If parameters are insufficient or types are incorrect, generate an error or warning.
***Provide default values and optional parameters:** For non-required parameters, provide reasonable default values. Additionally, use variable parameter lists like `varargin` to handle optional parameters, allowing users to specify these parameters as needed.
***Write clear and comprehensive documentation:** Clearly document all input parameters in the function documentation, including default values and optional parameters. Provide detailed explanations of parameter usage and impacts.
***Conduct comprehensive testing and validation:** Write test cases to verify the behavior of the function under different combinations of input parameters. Ensure that the function works as expected in all cases, including when input parameters are insufficient.
# Best Practices and Recommendations for Insufficient Input Parameters
### 6.1 Write Clear and Comprehensive Documentation
Clear documentation is crucial for preventing insufficient input parameters. The documentation should include the following information:
- The intended use and purpose of the function
- Description, type, and default value (if any) of each input parameter
- Behavior of the function when input parameters are insufficient
- Recommended strategies for handling insufficient input parameters
### 6.2 Use Robust Programming Techniques
Robust programming techniques help handle cases of insufficient input parameters. Here are some best practices:
- **Use exception handling:** Use `try-catch` blocks to catch exceptions for insufficient input parameters and provide meaningful error messages.
- **Use default values:** Specify default values for optional parameters to avoid cases of insufficient input parameters.
- **Use optional parameters:** Using optional parameters allows users to specify particular parameters while maintaining the flexibility of the function.
### 6.3 Conduct Comprehensive Testing and Validation
Comprehensive testing and validation are the last line of defense against insufficient input parameters. Testing should include the following:
- **Boundary value testing:** Test the behavior of the function near the boundaries of insufficient input parameters.
- **Negative testing:** Test the behavior of the function when invalid or unexpected input parameters are provided.
- **Integration testing:** Test the function when integrated with other modules or components and input parameters are insufficient.
By following these best practices and recommendations, the impact of insufficient input parameters on MATLAB functions can be significantly reduced, thereby enhancing the robustness and reliability of the code.
0
0
相关推荐





