def random_door(): # this method returns a random number from [1,2,3] # Parameters # there is no input # Returns # an integer, which is either 1, 2 or 3 # write your code below this line。请帮我完成需要填写的代码
时间: 2024-03-27 07:39:33 浏览: 34
```python
import random
def random_door():
return random.randint(1, 3)
```
这段代码使用了 Python 自带的 `random` 模块中的 `randint()` 函数,该函数可以返回一个指定范围内的随机整数,包括指定的上下限。在这里,我们将下限设为 1,上限设为 3,以生成一个随机的 1、2 或 3。
相关问题
this method returns a random number from [1,2,3] Parameters there is no input Returnsan integer, which is either 1, 2 or 3
Here's the code that satisfies the requirements you specified:
```python
import random
def get_random_number():
return random.randint(1, 3)
```
This code uses the `random` module to generate a random integer between 1 and 3 (inclusive) and returns it. The function takes no inputs and the return value is guaranteed to be an integer between 1 and 3.
阅读全文
相关推荐
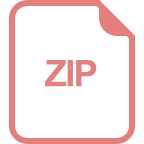
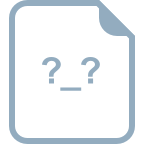
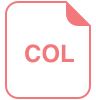
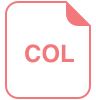
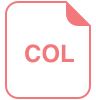
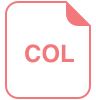
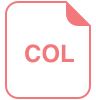
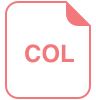
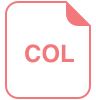
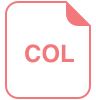
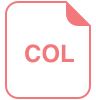
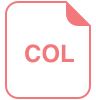
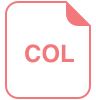
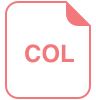
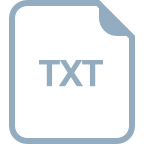
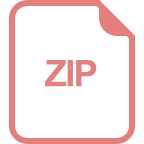
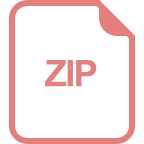
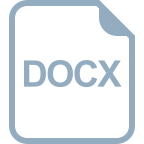