【Foundation】Signal Sampling and Quantization in MATLAB: Understanding the Sampling Theorem and Quantization Error
发布时间: 2024-09-14 05:41:29 阅读量: 49 订阅数: 85 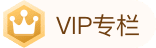
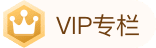
# 1. Signal Sampling and Quantization Overview
Signal sampling and quantization are two fundamental processes in digital signal processing that convert continuous-time, continuous-amplitude analog signals into discrete-time, discrete-amplitude digital signals. The sampling process takes analog signals and samples them at certain time intervals, while the quantization process discretizes the sampled signal values into a finite number of amplitude levels.
Sampling and quantization are crucial for digital signal processing as they allow analog signals to be processed, stored, and transmitted within digital systems. However, the sampling and quantization processes introduce errors, so careful consideration of sampling rates and quantization levels is necessary when designing digital signal processing systems.
# 2. Sampling Theorem and Sampling Rate Selection
### 2.1 Nyquist Sampling Theorem
The Nyquist Sampling Theorem is a fundamental theorem in the field of signal processing that specifies the relationship between the sampling rate and the signal bandwidth. The theorem states that to avoid aliasing during the sampling process, the sampling rate must be at least twice the highest frequency of the signal.
**Mathematical formula:**
```
f_s ≥ 2f_m
```
where:
* `f_s` is the sampling rate
* `f_m` is the highest frequency of the signal
### 2.2 Over-sampling and Under-sampling
**Over-sampling** refers to a sampling rate that is higher than the Nyquist sampling rate. Over-sampling can improve the quality of the sampled signal, reduce aliasing, but it also increases the amount of sampling data.
**Under-sampling** refers to a sampling rate that is lower than the Nyquist sampling rate. Under-sampling can cause aliasing in the sampled signal, resulting in distortion of the original signal.
### 2.3 Principles of Sampling Rate Selection
The selection of the sampling rate should be based on the following principles:
1. **Signal Bandwidth:** The sampling rate should be at least twice the highest frequency of the signal.
2. **Aliasing Tolerance:** If some degree of aliasing is acceptable, the sampling rate can be appropriately reduced.
3. **Data Volume:** A high sampling rate will generate a large amount of data, which should be considered in terms of data storage and processing capabilities.
4. **Hardware Limitations:** Some sampling devices may have limitations on the sampling rate.
**Code Block:**
```python
import numpy as np
import matplotlib.pyplot as plt
# Original signal
t = np.linspace(0, 1, 1000)
x = np.sin(2 * np.pi * 10 * t) + np.sin(2 * np.pi * 20 * t)
# Over-sampling
fs_over = 500
x_over = x[::int(1000 / fs_over)]
# Under-sampling
fs_under = 100
x_under = x[::int(1000 / fs_under)]
# Plot original signal and sampled signals
plt.plot(t, x, label="Original Signal")
plt.plot(t[::int(1000 / fs_over)], x_over, label="Over-sampling")
plt.plot(t[::int(1000 / fs_under)], x_under, label="Under-sampling")
plt.legend()
plt.show()
```
**Code Logic Analysis:**
* `np.linspace(0, 1, 1000)` generates 1000 sampling points uniformly distributed between 0 and 1.
* `np.sin(2 * np.pi * 10 * t) + np.sin(2 * np.pi * 20 * t)` generates an original signal containing two sine waves.
* `x_over = x[::int(1000 / fs_over)]` oversamples the original signal with a step size of `int(1000 / fs_over)`.
* `x_under = x[::int(1000 / fs_under)]` undersamples the original signal with a step size of `int(1000 / fs_under)`.
* `plt.plot()` plots the original and sampled signals.
**Parameter Description:**
* `fs_over`: Over-sampling rate
* `fs_under`: Under-sampling rate
# 3. Quantization Process and Quantization Error
### 3.1 Concept of Quantization and Quantization Levels
Quantization is the encoding process that converts continuous signal amplitude values into a finite number of discrete values. A quantizer divides the amplitude range of the continuous signal into multiple discrete quantization levels, with each level corresponding to a specific digital value.
The number of quantization levels determines the accuracy of the quantization. More quantization levels mean higher quantization accuracy and smaller quantization error. However, more quantization levels also mean more storage space and processing time are required.
### 3.2 Types and Effects of Quantization Error
Quantization error is an inevitable error that occurs during the quantization process, causing distortion of the signal. There are two main types of quantization errors:
- **Truncation Error:** The amplitude of the continuous signal is truncated to the nearest quantization level. The range of truncation error is [-LSB/2, LSB/2], where LSB is the quantizer's minimum quantization step.
- **Rounding Error:** The amplitude of the continuous signal is rounded to the nearest quantization level. The range of rounding error is [-LSB/4, LSB/4].
Quantization error affects the fidelity and signal-to-noise ratio (SNR) of the signal. The larger the quantization error, the lower the signal fidelity and SNR.
### 3.3 Assessment and Control of Quantization Error
To assess quantization error, the following indicators can be used:
- **Quantization Noise Power:** The average power of quantization error, measured in watts.
- **Signal-to-Noise Ratio (SNR):** The ratio of the original signal power to the quantization noise power, measured in decibels (dB).
To control quantization error, the following measures can be taken:
- **Increase Quantization Levels:** Increasing quantization levels can reduce quantization error, but it will also increase storage space and processing time.
- **Use Non-Uniform Quantization:** Allocate quantization levels to the non-uniform areas of the signal amplitude distribution to improve quantization accuracy.
- **Use Dithering Techniques:** Introducing random dithering during the quantization process can reduce the magnitude of quantization error.
```python
import numpy as np
# Continuous signal
signal = np.linspace(-1, 1, 1000)
# Quantization levels
levels = 8
# Quantizer
quantizer = np.round(signal / levels) * levels
# Quantization error
error = signal - quantizer
# Quantization noise power
noise_power = np.mean(error ** 2)
# Signal-to-Noise Ratio
snr = 10 * np.log10(np.mean(signal ** 2) / noise_power)
print(f"Quantization Noise Power: {noise_power}")
print(f"Signal-to-Noise Ratio: {snr}")
```
Code Logic:
1. Generate a continuous signal.
2. Set the number of quantization levels.
3. Quantize using the `np.round()` function.
4. Calculate the quantization error.
5. Calculate the quantization noise power.
6. Calculate the signal-to-noise ratio.
# 4. Practical Application of Signal Sampling and Quantization
### 4.1 Implementation of the Sampling Process
**Analog Signal Sampling**
Analog signal sampling uses an analog-to-digital converter (ADC) to convert a continuous-time signal into a discrete-time signal. The basic principle of an ADC is to compare the input signal with a set of known reference voltages and output a digital value proportional to the input signal.
**Sampling Process Implementation Code:**
```python
import numpy as np
def analog_sampling(signal, sampling_rate):
"""Analog signal sampling.
Args:
signal: Analog signal.
sampling_rate: Sampling rate.
Returns:
Sampled signal.
"""
# Create an ADC
adc = ADC()
# Sample the signal
sampled_signal = []
for sample in signal:
sampled_signal.append(adc.convert(sample))
return np.array(sampled_signal)
```
**Logic Analysis:**
* The `analog_sampling()` function takes the analog signal and sampling rate as parameters.
* The function creates an ADC object and uses the `convert()` method to convert each analog signal sample into a digital value.
* The sampled signal is stored in the `sampled_signal` list and returned as a NumPy array.
### 4.2 Implementation of the Quantization Process
**Quantization Process**
The quantization process converts the continuous-amplitude sampled signal into a digital signal with a finite number of discrete amplitudes. The quantizer maps the input signal to a set of discrete quantization levels and outputs the quantization level closest to the input signal.
**Quantization Process Implementation Code:**
```python
import numpy as np
def quantization(signal, num_levels):
"""Quantization process.
Args:
signal: Sampled signal.
num_levels: Number of quantization levels.
Returns:
Quantized signal.
"""
# Create a quantizer
quantizer = Quantizer(num_levels)
# Quantize the signal
quantized_signal = []
for sample in signal:
quantized_signal.append(quantizer.quantize(sample))
return np.array(quantized_signal)
```
**Logic Analysis:**
* The `quantization()` function takes the sampled signal and the number of quantization levels as parameters.
* The function creates a quantizer object and uses the `quantize()` method to map each sampled signal sample to the nearest quantization level.
* The quantized signal is stored in the `quantized_signal` list and returned as a NumPy array.
### 4.3 Effects of Sampling and Quantization on Signal Quality
Sampling and quantization processes introduce errors that affect signal quality.
**Sampling Error**
Sampling error is caused by converting a continuous-time signal into a discrete-time signal. The higher the sampling rate, the smaller the sampling error.
**Quantization Error**
Quantization error is caused by converting a continuous-amplitude signal into a finite number of discrete amplitudes. The more quantization levels, the smaller the quantization error.
**Effects of Sampling and Quantization on Signal Quality**
Sampling and quantization errors affect the fidelity, spectrum, and dynamic range of the signal.
**Effects on Spectrum:** Sampling error can introduce aliasing, where high-frequency components of the signal appear in the low-frequency components. Quantization error can introduce quantization noise, adding unnecessary noise to the signal.
**Effects on Dynamic Range:** Quantization error limits the dynamic range of the signal, which is the difference between the maximum and minimum values of the signal.
**Table 4.1: Effects of Sampling and Quantization on Signal Quality**
| Error Type | Effect |
|---|---|
| Sampling Error | Aliasing |
| Quantization Error | Quantization Noise |
| Sampling and Quantization Errors | Reduced Fidelity, Spectrum Distortion, Limited Dynamic Range |
**mermaid Flowchart:**
```mermaid
graph LR
subgraph Sampling Process
Sampler --> Sampled Signal
end
subgraph Quantization Process
Quantizer --> Quantized Signal
end
subgraph Effects of Sampling and Quantization on Signal Quality
Sampling Error --> Aliasing
Quantization Error --> Quantization Noise
Sampling and Quantization Errors --> Reduced Fidelity
Sampling and Quantization Errors --> Spectrum Distortion
Sampling and Quantization Errors --> Limited Dynamic Range
end
```
# 5. Application of Signal Sampling and Quantization in MATLAB
### 5.1 Using S***
***mon sampling functions include:
```
>> x = linspace(0, 2*pi, 1000);
>> y = sin(x);
>> fs = 1000;
>> t = linspace(0, 2*pi, length(y)/fs);
```
- `linspace`: Generates uniformly spaced sampling points.
- `sin`: Generates a sine wave signal.
- `fs`: Sampling rate.
- `t`: Sampling time points.
### 5.2 U***
***mon quantization functions include:
```
>> y_quantized = round(y);
>> y_quantized = quantize(y, -1:0.1:1);
```
- `round`: Rounds to the nearest integer.
- `quantize`: Quantizes according to specified quantization levels.
### 5.3 Effects of Sampling and Quantization on MATLAB Signal Processing
Sampling and quantization are indispensable steps in signal processing. Their effects on MATLAB signal processing are mainly reflected in the following aspects:
- **Sampling Rate Selection:** Too low a sampling rate can cause aliasing, while too high a rate can lead to wasted computational resources.
- **Quantization Accuracy:** Too low quantization accuracy can cause quantization errors, affecting signal quality.
- **Effects on Spectrum:** Sampling and quantization can introduce new frequency components, affecting the signal's spectral characteristics.
- **Effects on Time-domain Signals:** Sampling and quantization can change the time-domain waveform of the signal, leading to distortion.
0
0
相关推荐








