【Practical Exercise】Implementing Basic Digital Filter Design with MATLAB
发布时间: 2024-09-14 06:43:54 阅读量: 41 订阅数: 83 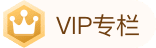
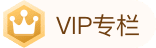
# 2.1 Low-Pass Filter Design
A low-pass filter is one that allows low-frequency signals to pass through while attenuating signals with higher frequencies. In MATLAB, the `butter` function can be used to design a Butterworth low-pass filter, with the following syntax:
```
[b, a] = butter(n, Wn, 'low')
```
Where:
* `n`: Filter order
* `Wn`: Normalized cutoff frequency (0-1)
* `'low'`: Specifies the type of filter as low-pass
For instance, to design a Butterworth low-pass filter with an order of 5 and a cutoff frequency of 0.2, the following code can be used:
```
[b, a] = butter(5, 0.2, 'low');
```
# 2. Filter Design Practice
### 2.1 Low-Pass Filter Design
A low-pass filter permits low-frequency signals to pass and attenuates high-frequency signals. In MATLAB, low-pass filters can be designed using the `butter` and `cheby1` functions.
#### 2.1.1 Butterworth Filter Design
Butterworth filters feature a flat passband response and a monotonic roll-off in the stopband. The `butter` function can be used to design Butterworth low-pass filters:
```matlab
[b, a] = butter(N, Wn); % N is the order, Wn is the cutoff frequency
```
Where:
* `b`: Numerator polynomial coefficients of the filter
* `a`: Denominator polynomial coefficients of the filter
* `N`: Filter order
* `Wn`: Cutoff frequency (normalized frequency between 0 and 1)
**Code Logic Analysis:**
* The `butter` function employs the Butterworth filter design technique.
* The `N` parameter specifies the order of the filter, determining the filter's steepness and passband attenuation.
* The `Wn` parameter specifies the filter's cutoff frequency, which defines the boundary between the passband and stopband.
#### 2.1.2 Chebyshev Filter Design
Chebyshev filters have steeper roll-off in the stopband compared to Butterworth filters but exhibit a non-flat passband response. The `cheby1` function can be used to design Chebyshev low-pass filters:
```matlab
[b, a] = cheby1(N, Rp, Wn); % N is the order, Rp is the passband ripple (in dB), Wn is the cutoff frequency
```
Where:
* `b`: Numerator polynomial coefficients of the filter
* `a`: Denominator polynomial coefficients of the filter
* `N`: Filter order
* `Rp`: Passband ripple (in dB)
* `Wn`: Cutoff frequency (normalized frequency between 0 and 1)
**Code Logic Analysis:**
* The `cheby1` function uses the Chebyshev filter design method.
* The `N` parameter specifies the order of the filter, determining the filter's steepness and passband attenuation.
* The `Rp` parameter specifies the filter's passband ripple, which defines the maximum attenuation within the passband.
* The `Wn` parameter specifies the filter's cutoff frequency, which defines the boundary between the passband and stopband.
### 2.2 High-Pass Filter Design
A high-pass filter allows high-frequency signals to pass through while attenuating low-frequency signals. In MATLAB, high-pass filters can be designed using the `butter` and `cheby2` functions.
#### 2.2.1 Butterworth Filter Design
The `butter` function can be used to design Butterworth high-pass filters:
```matlab
[b, a] = butter(N, Wn, 'high'); % N is the order, Wn is the cutoff frequency
```
Where:
* `b`: Numerator polynomial coefficients of the filter
* `a`: Denominator polynomial coefficients of the filter
* `N`: Filter order
* `Wn`: Cutoff frequency (normalized frequency between 0 and 1)
**Code Logic Analysis:**
* The `butter` function employs the Butterworth filter design method.
* The `N` parameter specifies the order of the filter, determining the filter's steepness and passband attenuation.
* The `Wn` parameter specifies the filter's cutoff frequency, which defines the boundary between the passband and stopband.
* The `'high'` parameter specifies that the filter type is a high-pass filter.
#### 2.2.2 Chebyshev Filter Design
The `cheby2` function can be used to design Chebyshev high-pass filters:
```matlab
[b, a] = cheby2(N, Rs, Wn); % N is the order, Rs is the stopband ripple (in dB), Wn is the cutoff frequency
```
Where:
* `b`: Numerator polynomial coefficients of the filter
* `a`: Denominator polynomial coefficients of the filter
* `N`: Filter order
* `Rs`: Stopband ripple (in dB)
* `Wn`: Cutoff frequency (normalized frequency between 0 and 1)
**Code Logic Analysis:**
* The `cheby2` function uses the Chebyshev filter design method.
* The `N` parameter specifies the order of th
0
0
相关推荐









