# 打开一个文件 scales = ["服务过程","管理过程","生产过程","研发过程"] results = [] for s in scales: results.append({}) f = open("../%s.txt"%s, encoding = "utf-8") string = f.readlines() words = string[0].split("、") for w in words: results[-1][w] = [] for i in range(10): f = open("../年报/%d.txt.txt"%(i+2011), "r",encoding='utf-8') target = "" string = f.readlines() for line in string: target += line target = target.replace(" ","") for j in range(len(scales)): fkey = open("../%s.txt"%scales[j], encoding = "utf-8") s = fkey.readlines() words = s[0].split("、") for w in range(len(words)): num = target.count(words[w]) results[j][words[w]].append(num) for i in range(len(results)): r = pd.DataFrame(results[i]) r.to_excel("%s_词频统计.xlsx"%scales[i],index_label = ["2011","2012","2013","2014","2015","2016","2017","2018","2019","2020"])
时间: 2023-07-21 09:04:12 浏览: 99
你的代码看起来是用来读取一些文件,并统计其中关键词的词频,然后将结果保存到Excel文件中。这个代码有几个问题需要注意一下。
首先,在打开文件时,最好使用上下文管理器(`with`语句)来确保文件能够正确关闭,以避免资源泄漏。这样可以替代你使用`open()`打开文件的方式。类似于这样:
```python
with open("../%s.txt" % s, encoding="utf-8") as f:
string = f.readlines()
```
其次,在统计关键词的词频时,你可以使用Python的`collections.Counter`类来简化代码。例如:
```python
from collections import Counter
# ...
for w in range(len(words)):
counter = Counter(target)
num = counter[words[w]]
results[j][words[w]].append(num)
```
最后,你需要导入`pandas`库,因为你的代码中使用了`pd.DataFrame()`和`to_excel()`方法。确保在代码开头添加`import pandas as pd`。
希望这些修改对你有帮助!如果你还有其他问题,请随时提出。
相关问题
解释代码:int post_process(int8_t* input0, int8_t* input1, int8_t* input2, int model_in_h, int model_in_w, float conf_threshold, float nms_threshold, float scale_w, float scale_h, std::vector<int32_t>& qnt_zps, std::vector<float>& qnt_scales, detect_result_group_t* group) { static int init = -1; if (init == -1) { int ret = 0; ret = loadLabelName(LABEL_NALE_TXT_PATH, labels); if (ret < 0) { return -1; } init = 0; } memset(group, 0, sizeof(detect_result_group_t)); std::vector<float> filterBoxes; std::vector<float> objProbs; std::vector<int> classId; // stride 8 int stride0 = 8; int grid_h0 = model_in_h / stride0; int grid_w0 = model_in_w / stride0; int validCount0 = 0; validCount0 = process(input0, (int*)anchor0, grid_h0, grid_w0, model_in_h, model_in_w, stride0, filterBoxes, objProbs, classId, conf_threshold, qnt_zps[0], qnt_scales[0]); // stride 16 int stride1 = 16; int grid_h1 = model_in_h / stride1; int grid_w1 = model_in_w / stride1; int validCount1 = 0; validCount1 = process(input1, (int*)anchor1, grid_h1, grid_w1, model_in_h, model_in_w, stride1, filterBoxes, objProbs, classId, conf_threshold, qnt_zps[1], qnt_scales[1]); // stride 32 int stride2 = 32; int grid_h2 = model_in_h / stride2; int grid_w2 = model_in_w / stride2; int validCount2 = 0; validCount2 = process(input2, (int*)anchor2, grid_h2, grid_w2, model_in_h, model_in_w, stride2, filterBoxes, objProbs, classId, conf_threshold, qnt_zps[2], qnt_scales[2]); int validCount = validCount0 + validCount1 + validCount2; // no object detect if (validCount <= 0) { return 0; } std::vector<int> indexArray; for (int i = 0; i < validCount; ++i) { indexArray.push_back(i); } quick_sort_indice_inverse(objProbs, 0, validCount - 1, indexArray); std::set<int> class_set(std::begin(classId), std::end(classId)); for (auto c : class_set) { nms(validCount, filterBoxes, classId, indexArray, c, nms_threshold); } int last_count = 0; group->count = 0; /* box valid detect target */ for (int i = 0; i < validCount; ++i) { if (indexArray[i] == -1 || last_count >= OBJ_NUMB_MAX_SIZE) { continue; } int n = indexArray[i]; float x1 = filterBoxes[n * 4 + 0]; float y1 = filterBoxes[n * 4 + 1]; float x2 = x1 + filterBoxes[n * 4 + 2]; float y2 = y1 + filterBoxes[n * 4 + 3]; int id = classId[n]; float obj_conf = objProbs[i]; group->results[last_count].box.left = (int)(clamp(x1, 0, model_in_w) / scale_w); group->results[last_count].box.top = (int)(clamp(y1, 0, model_in_h) / scale_h); group->results[last_count].box.right = (int)(clamp(x2, 0, model_in_w) / scale_w); group->results[last_count].box.bottom = (int)(clamp(y2, 0, model_in_h) / scale_h); group->results[last_count].prop = obj_conf; char* label = labels[id]; strncpy(group->results[last_count].name, label, OBJ_NAME_MAX_SIZE); // printf("result %2d: (%4d, %4d, %4d, %4d), %s\n", i, group->results[last_count].box.left, // group->results[last_count].box.top, // group->results[last_count].box.right, group->results[last_count].box.bottom, label); last_count++; } group->count = last_count; return 0; }
这段代码实现了目标检测的后处理函数,对模型输出的特征图进行处理,并生成检测结果。具体解释如下:
函数参数:
- `input0`、`input1`、`input2`:三个特征图的指针,分别对应 stride 为 8、16、32 的特征图。
- `model_in_h`、`model_in_w`:输入模型的高度和宽度。
- `conf_threshold`:目标置信度的阈值。
- `nms_threshold`:非极大值抑制的阈值。
- `scale_w`、`scale_h`:图像的缩放因子。
- `qnt_zps`、`qnt_scales`:量化参数。
- `group`:存储检测结果的指针。
函数功能:
1. 首先,检查是否需要初始化标签名,如果是第一次调用函数,则加载标签名并保存在全局变量 `labels` 中。
2. 初始化存储检测结果的 `group` 结构体,并将其内存清零。
3. 创建用于存储过滤后的检测框位置和大小的向量 `filterBoxes`,以及存储目标置信度和类别编号的向量 `objProbs` 和 `classId`。
4. 对 stride 为 8 的特征图进行处理,计算有效目标数目,并将结果保存在 `validCount0` 中。
5. 对 stride 为 16 和 32 的特征图进行类似的处理,计算有效目标数目并分别保存在 `validCount1` 和 `validCount2` 中。
6. 计算总的有效目标数目 `validCount`,如果没有检测到目标,则直接返回。
7. 创建一个索引数组 `indexArray`,用于排序和非极大值抑制操作。
8. 使用快速排序算法 `quick_sort_indice_inverse` 对目标置信度 `objProbs` 进行降序排序,并记录索引的变化情况。
9. 创建一个集合 `class_set`,用于存储所有出现的类别编号。
10. 针对每个类别对目标框进行非极大值抑制操作,剔除重叠度较高的重复框,保留置信度最高的框。
11. 初始化最终检测结果计数器 `last_count` 和 `group->count`。
12. 遍历排序后的索引数组 `indexArray`,获取每个目标框的位置、大小、类别编号和置信度,并进行一些后处理操作。
13. 将检测结果转换为图像坐标,并保存在 `group->results` 中。
14. 更新最终检测结果计数器 `last_count`。
15. 将最终的检测结果数目保存在 `group->count` 中。
16. 返回 0 表示处理成功。
通过这样的处理过程,可以从模型输出的特征图中提取出有效的目标检测结果,并进行非极大值抑制操作,最终生成包含检测框位置、大小、类别和置信度的结果。
对上述代码进行如下修改,是否改变基本功能:tatic int process(int8_t* input, int point_cnt, int height, int width, int stride, std::vector<float>& boxes, std::vector<float>& objProbs, std::vector<int>& classId, float threshold, int32_t zp, float scale) { int validCount = 0; float thres = unsigmoid(threshold); int8_t thres_i8 = qnt_f32_to_affine(thres, zp, scale); for (int a = 0; a < point_cnt; a++){ int8_t maxClassProbs = 0; int maxClassId = 0; for (int k = 1; k < OBJ_CLASS_NUM; ++k) { int8_t prob = input[(3+k) * point_cnt + a]; if (prob > maxClassProbs) { maxClassId = k; maxClassProbs = prob; } } if (maxClassProbs >= thres_i8) { int8_t rx = input[0 * point_cnt + a]; int8_t ry = input[1 * point_cnt + a]; int8_t rw = input[2 * point_cnt + a]; int8_t rh = input[3 * point_cnt + a]; float box_x = sigmoid(deqnt_affine_to_f32(rx, zp, scale)) * 2.0 - 0.5; float box_y = sigmoid(deqnt_affine_to_f32(ry, zp, scale)) * 2.0 - 0.5; float box_w = sigmoid(deqnt_affine_to_f32(rw, zp, scale)) * 2.0; float box_h = sigmoid(deqnt_affine_to_f32(rh, zp, scale)) * 2.0; objProbs.push_back(sigmoid(deqnt_affine_to_f32(maxClassProbs, zp, scale))); classId.push_back(maxClassId); validCount++; boxes.push_back(box_x); boxes.push_back(box_y); boxes.push_back(box_w); boxes.push_back(box_h); } } return validCount; } int post_process(int8_t* input0, int model_in_h, int model_in_w, float conf_threshold, float nms_threshold, float scale_w, float scale_h, std::vector<int32_t>& qnt_zps, std::vector<float>& qnt_scales, detect_result_group_t* group) { static int init = -1; if (init == -1) { int ret = 0; ret = loadLabelName(LABEL_NALE_TXT_PATH, labels); if (ret < 0) { return -1; } init = 0; } memset(group, 0, sizeof(detect_result_group_t)); std::vector<float> filterBoxes; std::vector<float> objProbs; std::vector<int> classId; // stride 6 int stride0 = 4 + OBJ_CLASS_NUM; int point_cnt = 8400; int validCount0 = 0; validCount0 = process(input0, point_cnt, model_in_h, model_in_w, stride0, filterBoxes, objProbs, classId, conf_threshold, qnt_zps[0], qnt_scales[0]); int validCount = validCount0; // no object detect if (validCount <= 0) { return 0; } std::vector<int> indexArray; for (int i = 0; i < validCount; ++i) { indexArray.push_back(i); } quick_sort_indice_inverse(objProbs, 0, validCount - 1, indexArray); std::set<int> class_set(std::begin(classId), std::end(classId)); for (auto c : class_set) { nms(validCount, filterBoxes, classId, indexArray, c, nms_threshold); } int last_count = 0; group->count = 0; /* box valid detect target */ for (int i = 0; i < validCount; ++i) { if (indexArray[i] == -1 || last_count >= OBJ_NUMB_MAX_SIZE) { continue; } int n = indexArray[i]; float x1 = filterBoxes[n * 4 + 0]; float y1 = filterBoxes[n * 4 + 1]; float x2 = x1 + filterBoxes[n * 4 + 2]; float y2 = y1 + filterBoxes[n * 4 + 3]; int id = classId[n]; float obj_conf = objProbs[i]; group->results[last_count].box.left = (int)(clamp(x1, 0, model_in_w) / scale_w); group->results[last_count].box.top = (int)(clamp(y1, 0, model_in_h) / scale_h); group->results[last_count].box.right = (int)(clamp(x2, 0, model_in_w) / scale_w); group->results[last_count].box.bottom = (int)(clamp(y2, 0, model_in_h) / scale_h); group->results[last_count].prop = obj_conf; char* label = labels[id]; strncpy(group->results[last_count].name, label, OBJ_NAME_MAX_SIZE); // printf("result %2d: (%4d, %4d, %4d, %4d), %s\n", i, group->results[last_count].box.left, // group->results[last_count].box.top, // group->results[last_count].box.right, group->results[last_count].box.bottom, label); last_count++; } group->count = last_count; return 0; }
对于第一个修改的代码块 `static int process(int8_t* input, int point_cnt, int height, int width, int stride,
std::vector<float>& boxes, std::vector<float>& objProbs, std::vector<int>& classId, float threshold,
int32_t zp, float scale)`,基本功能并没有改变。该函数仍然实现了目标检测的后处理,根据输入的 int8_t 类型指针和相关参数,提取有效的目标检测结果,并将检测框的位置、大小、目标置信度和类别编号存储在相应的向量中。
第二个修改的代码块 `int post_process(int8_t* input0, int model_in_h, int model_in_w, float conf_threshold,
float nms_threshold, float scale_w, float scale_h, std::vector<int32_t>& qnt_zps,
std::vector<float>& qnt_scales, detect_result_group_t* group)`,基本功能也没有改变。该函数仍然实现了目标检测的后处理,根据输入的特征图和相关参数,提取有效的目标检测结果,并将检测框的位置、大小、目标置信度和类别信息存储在 `detect_result_group_t` 结构体中。
两个修改的代码块主要对输入参数进行了调整,其中 `point_cnt` 表示检测点的数量,通过调整 `stride0` 的计算方式来确定。这些修改可能是为了适应不同的检测模型或数据集,但并没有改变基本的目标检测后处理逻辑。
阅读全文
相关推荐
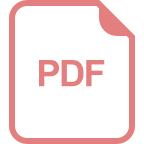
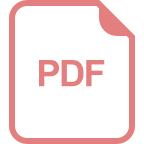
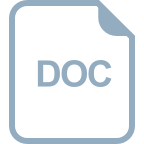
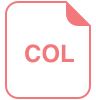
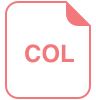
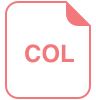
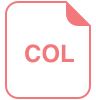
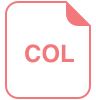
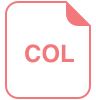
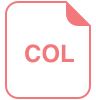
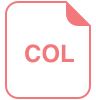
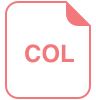
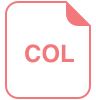
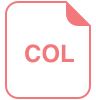
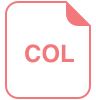
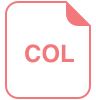
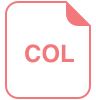