Empowering All Sectors: A Detailed Explanation of OpenCV Machine Vision Applications, from Industrial Automation to Medical Imaging
发布时间: 2024-09-15 10:45:05 阅读量: 6 订阅数: 13 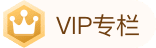
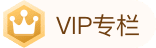
# Empowering Industries: A Detailed Exploration of OpenCV Applications in Machine Vision, from Industrial Automation to Medical Imaging
# 1. Overview of OpenCV Machine Vision
OpenCV (Open Source Computer Vision Library) is an open-source library of computer vision algorithms, offering a wide range of image processing and analysis techniques. It is widely used in industries such as industrial automation, medical imaging, and robotic navigation.
The advantages of OpenCV lie in its cross-platform compatibility, ease of use, and a rich algorithm library. It supports various programming languages, such as C++, Python, and Java, and provides intuitive APIs that facilitate a quick start for developers. Moreover, OpenCV's algorithm library covers image processing, feature extraction, object recognition, machine learning, and more, offering comprehensive computer vision solutions.
# 2.1 Fundamentals of Image Processing
### 2.1.1 Image Formats and Conversion
**Image Formats**
***mon formats include:
- **Bitmap (BMP)**: An uncompressed format with larger file size.
- **JPEG (Joint Photographic Experts Group)**: A lossy compression format suitable for natural images.
- **PNG (Portable Network Graphics)**: A lossless compression format ideal for images with lines and text.
- **TIFF (Tagged Image File Format)**: Suitable for high-quality images and larger in size.
**Image Conversion**
Image conversion involves changing the format from one to another. OpenCV provides various functions for image conversion, such as:
```python
import cv2
# Convert BGR image to HSV image
hsv_image = cv2.cvtColor(bgr_image, cv2.COLOR_BGR2HSV)
# Convert grayscale image to binary image
binary_image = cv2.threshold(gray_image, 127, 255, cv2.THRESH_BINARY)[1]
```
### 2.1.2 Image Enhancement and Filtering
**Image Enhancement**
Image enhancement techniques aim to improve the visual quality of images, ***mon enhancement techniques include:
- **Contrast Enhancement**: Adjusts the difference between light and dark areas in an image.
- **Brightness Adjustment**: Changes the overall brightness of the image.
- **Histogram Equalization**: Redistributes pixel values in the image to increase contrast.
**Filtering**
Filtering is used to remove noise and blur from images, ***mon filters include:
- **Mean Filtering**: Calculates the average of the neighboring pixels and replaces the central pixel with the average.
- **Median Filtering**: Calculates the median of the neighboring pixels and replaces the central pixel with the median.
- **Gaussian Filtering**: Uses a Gaussian kernel for convolution to smooth the image and remove noise.
```python
import cv2
# Apply mean filtering
mean_filtered_image = cv2.blur(image, (5, 5))
# Apply median filtering
median_filtered_image = cv2.medianBlur(image, 5)
# Apply Gaussian filtering
gaussian_filtered_image = cv2.GaussianBlur(image, (5, 5), 0)
```
# 3. Applications of OpenCV in Industrial Automation
### 3.1 Visual Inspection and Quality Control
An important application of OpenCV in industrial automation is visual inspection and quality control. By leveraging computer vision techniques, OpenCV can assist in automating product defect detection, product classification, and sorting tasks.
#### 3.1.1 Product Defect Detection
Product defect detection is a critical task in industrial automation. OpenCV can be used to train computer vision models to identify defects in products, such as scratches, dents, or discoloration. These models can be deployed on production lines to inspect products in real-time and identify defects.
```python
import cv2
# Load a pre-trained defect detection model
model = cv2.dnn.readNetFromCaffe("deploy.prototxt.txt", "model.caffemodel")
# Load the image to be inspected
image = cv2.imread("product.jpg")
# Preprocess the image
image = cv2.resize(image, (300, 300))
image = cv2.cvtColor(image, cv2.COLOR_BGR2RGB)
# Run the model for defect detection
detections = model.detect(image)
# Iterate over the detection results and draw defect bounding boxes
for detection in detections:
x1, y1, x2, y2 = detection[2][0:4].astype(int)
cv2.rectangle(image, (x1, y1), (x2, y2), (0, 0, 255), 2)
# Display the detection results
cv2.imshow("Defect Detection", image)
cv2.waitKey(0)
```
#### 3.1.2 Product Classification and Sorting
Product classification and sorting are other critical tasks in industrial au
0
0
相关推荐
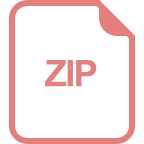
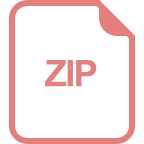





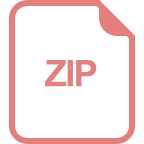
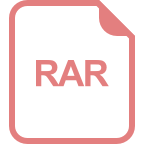
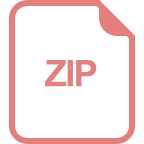