Capturing Motion Information in Images: A Detailed Explanation of OpenCV Motion Estimation Algorithms, from Optical Flow to Lucas-Kanade
发布时间: 2024-09-15 10:39:13 阅读量: 32 订阅数: 24 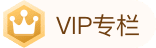
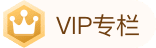
# 1. Overview of Image Motion Estimation Algorithms
Image motion estimation algorithms aim to estimate the motion parameters of moving objects from a sequence of consecutive images. They are widely used in the fields of computer vision and video analysis, such as motion tracking, video compression, and object detection.
Motion estimation algorithms are typically based on the optical flow equation, which describes the rate at which pixels move over time in an image. By solving the optical flow equation, we can obtain the motion vectors of moving objects in the image.
The optical flow method is one of the most commonly used methods in image motion estimation algorithms. It estimates optical flow by minimizing pixel brightness differences. There are many different algorithm implementations of the optical flow method, the most popular of which include the Horn-Schunck algorithm and the Lucas-Kanade algorithm.
# 2. Principles and Practice of the Optical Flow Method
### 2.1 Basic Concepts and Mathematical Models of the Optical Flow Method
#### 2.1.1 Optical Flow Equation
The optical flow method is a computer vision technique used to estimate pixel motion in image sequences. Its basic assumption is that adjacent pixels in the image move along their motion trajectories in consecutive frames, i.e., the changes in pixel grayscale values over time and space satisfy the following optical flow equation:
```
I(x, y, t) = I(x + dx, y + dy, t + dt)
```
Where:
- `I(x, y, t)` represents the pixel grayscale value at the coordinates `(x, y)` in the image at time `t`
- `(dx, dy)` represents the motion displacement of the pixel over the time interval `dt`
#### 2.1.2 Optical Flow Constraints
Since changes in image brightness over time may be caused by motion or changes in illumination, ***mon optical flow constraints include:
- **Brightness Constancy Constraint:** Assumes that the pixel grayscale value remains constant over time, i.e., `I(x, y, t) = I(x + dx, y + dy, t + dt)`.
- **Gradient Constancy Constraint:** Assumes that the pixel gradient remains constant over time, i.e., `∇I(x, y, t) = ∇I(x + dx, y + dy, t + dt)`.
### 2.2 Algorithm Implementation of the Optical Flow Method
#### 2.2.1 Horn-Schunck Algorithm
The Horn-Schunck algorithm is an optical flow algorithm based on the brightness constancy constraint. Its principle is to estimate the optical flow field by minimizing the following energy function:
```
E(u, v) = ∫∫(I(x, y, t) - I(x + u, y + v, t + dt))^2 + λ(∇u)^2 + λ(∇v)^2) dx dy
```
Where:
- `(u, v)` represents the motion displacement of the pixel over the time interval `dt`
- `λ` is the regularization parameter, which controls the smoothness
This energy function is minimized through an iterative optimization algorithm, thus obtaining the optical flow field.
#### 2.2.2 Lucas-Kanade Algorithm
The Lucas-Kanade algorithm is an optical flow algorithm based on the gradient constancy constraint. Its principle is to estimate the optical flow field by minimizing the following objective function:
```
E(u, v) = ∫∫(I(x, y, t) - I(x + u, y + v, t + dt))^2 dx dy
```
This objective function is minimized through an iterative optimization algorithm, thus obtaining the optical flow field.
**Code Block:**
```python
import cv2
# Calculate optical flow
flow = cv2.calcOpticalFlowFarneback(prev_frame, curr_frame, None, 0.5, 3, 15, 3, 5, 1.2, 0)
# Visualize optical flow
hue = np.arctan2(flow[..., 1], flow[..., 0]) / np.pi
mag = cv2.norm(flow, axis=2)
rgb = np.concatenate((hue[:, :, np.newaxis], mag[:, :, np.newaxis], np.ones_like(hue[:, :, np.newaxis])), axis=2)
hsv = cv2.cvtColor(rgb, cv2.COLOR_RGB2HSV)
flow_image = cv2.cvtColor(hsv, cv2.COLOR_HSV2BGR)
```
**Logical Analysis:**
This code block uses the `calcOpticalFlowFarneback` function from OpenCV to calculate the optical flow. The function parameters include:
- `prev_frame`: Previous frame image
- `curr_frame`: Current frame image
- `None`: Do not use the pyramid algorithm
- `0.5`: Step size of moving pixels
- `3`: Size of the search window
- `15`: Number
0
0
相关推荐
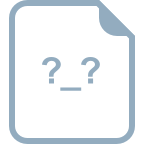
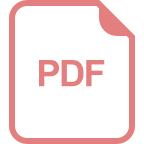
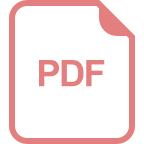
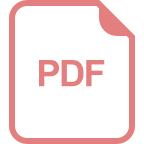
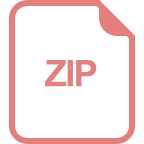
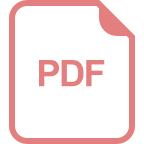
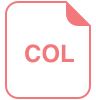
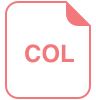
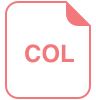