Parallelization of MATLAB Functions: Enhancing Function Performance with Multi-core Processors
发布时间: 2024-09-14 12:16:23 阅读量: 32 订阅数: 37 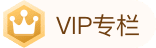
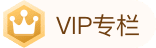
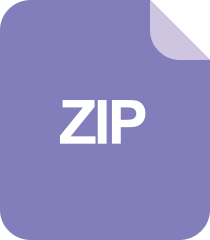
pm代码matlab-Parallelization_Workshop:并行化_研讨会
# 1. Basic Theory of MATLAB Function Parallelization
## 1.1 Concept of Parallel Computing
Parallel computing is a technique that utilizes multiple processing units to perform tasks simultaneously to enhance computational efficiency. It achieves this by breaking a large task into smaller sub-tasks and executing these in parallel on multiple processing units.
## 1.2 Parallelization in MATLAB
MATLAB provides a Parallel Computing Toolbox, enabling you to easily parallelize MATLAB functions. The toolbox offers a variety of functions and tools for creating and managing parallel programs.
# 2. Programming Techniques for MATLAB Function Parallelization
## 2.1 Parallel Programming Models
### 2.1.1 SPMD Model
The SPMD (Single Program Multiple Data) model is a parallel programming model in which all processes execute the same code, but operate on different data. SPMD is suitable for data parallel problems where data can be divided into multiple independent parts, with each process handling one part.
**Advantages:**
* Programming is simple because all processes execute the same code.
* Data parallel problems are easy to decompose.
**Disadvantages:**
* Parallelizing control flows can be difficult.
* Load balancing can be a challenge.
**Code Example:**
```matlab
% Create a parallel pool
parpool;
% Define a function to be executed in parallel
myFunction = @(x) x^2;
% Create a data array
data = rand(100000, 1);
% Execute the function in parallel
parfor i = 1:length(data)
data(i) = myFunction(data(i));
end
% Close the parallel pool
delete(gcp);
```
**Logical Analysis:**
* The `parpool` function creates a pool of multiple workers.
* The `myFunction` function defines the computation to be executed in parallel.
* The `parfor` loop applies the `myFunction` function in parallel to each element of the `data` array.
* The `delete(gcp)` function closes the parallel pool.
### 2.1.2 MPI Model
The MPI (Message Passing Interface) model is a parallel programming model where processes communicate through explicit message passing. MPI is suitable for parallel problems that require complex control flows or inter-process communication.
**Advantages:**
* Provides fine-grained control over parallelization.
* Suitable for various parallel problems.
**Disadvantages:**
* Programming can be complex, requiring explicit message passing.
* Debugging can be difficult.
**Code Example:**
```matlab
% Create an MPI parallel environment
mpi_init;
% Get the number of processes
num_procs = mpi_comm_size(MPI_COMM_WORLD);
% Get the process rank
my_rank = mpi_comm_rank(MPI_COMM_WORLD);
% Parallel computation
if my_rank == 0
% The master process sends data to other processes
data = rand(100000, 1);
for i = 1:num_procs-1
mpi_send(data, i, 0, MPI_COMM_WORLD);
end
else
% Other processes receive data and perform computation
data = mpi_recv(0, my_rank, 0, MPI_COMM_WORLD);
data = data.^2;
% Send the results back to the master process
mpi_send(data, 0, 0, MPI_COMM_WORLD);
end
% The master process collects results
if my_rank == 0
for i = 1:num_procs-1
data = mpi_recv(i, 0, 0, MPI_COMM_WORLD);
% ...
end
end
% Terminate the MPI parallel environment
mpi_finalize;
```
**Logical Analysis:**
* The `mpi_init` function initializes the MPI parallel environment.
* The `mpi_comm_size` function gets the number of processes.
* The `mpi_comm_rank` function gets the process rank.
* The master process (rank 0) uses the `mpi_send` function to send data to other processes.
* Other processes use the `mpi_recv` function to receive data, perform computation, and then send the results back to the master process.
* The master process uses `mpi_recv` to collect results.
* The `mpi_finalize` function terminates the MPI parallel environment.
# 3. Practical Application of MATLAB Function Parallelization
### 3.1 Parallelization of Numerical Computation
Numerical computation is one of the most widely parallelized fields in MATLAB, including matrix operations, optimization algorithms, etc.
#### 3.1.1 Matrix Operations
Matrix operations are crucial in numerical computation, and MATLAB offers a wealth of parallel matrix operation functions, such as `parfor` and `spmd`.
**Code Block 1: Parallel Matrix Multiplication**
```matlab
% Create two matrices
A = randn(1000, 1000);
B = randn(1000, 1000);
% Parallel computation of matrix multiplication
C = zeros(1000, 1000);
parfor i = 1:1000
for j = 1:1000
C(i, j) = A(i, :) * B(:, j);
end
end
```
**Logical Analysis:**
This code block uses the `parfor` loop to parallelize the calculation of elements in matrix `C`. The outer loop parallelizes the rows, and the inner loop parallelizes the columns, thus achieving parallelization of matrix multiplication.
#### 3.1.2 Optimization Algorithms
Optimization algorithms are widely used in solving complex problems. MATLAB provides a parallel optimization toolbox that supports the parallelization of various optimization algorithms.
**Code Block 2: Parallel Particle Swarm Optimization**
```matlab
% Define the particle swarm optimization problem
problem = @myObjectiveFunction;
% Perform parallel particle swarm optimization
options = optimoptions('particleswarm', 'SwarmSize', 100, 'MaxIterations', 100, 'UseParallel', true);
[x, fval] = part
```
0
0
相关推荐







