【Advanced】MATLAB Data Acquisition Toolbox: Control Systems Toolbox User Guide
发布时间: 2024-09-13 16:19:23 阅读量: 8 订阅数: 26 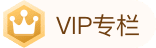
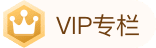
# Introduction to the Control Systems Toolbox for MATLAB
The Control Systems Toolbox is a powerful suite of tools in MATLAB designed for the design, analysis, and simulation of control systems. It offers a wide range of functionalities, including data acquisition, data analysis, visualization, system modeling, simulation, control law design, and performance analysis. This toolbox is widely used across various industries, including aerospace, automotive, industrial automation, and robotics technology.
# Key Features of the Data Acquisition Toolbox
## 2.1 Data Acquisition and Processing
### 2.1.1 Connection and Configuration of Data Acquisition Devices
The Control Systems Toolbox provides tools for connecting and configuring various data acquisition devices, such as data acquisition cards, sensors, and actuators. The toolbox includes functions for connecting and configuring these devices, such as `daq.createSession`, `daq.addAnalogInputChannel`, and `daq.startBackground`.
**Code Block:**
```matlab
daqSession = daq.createSession('ni');
daqSession.addAnalogInputChannel('Dev1', 'ai0', 'Voltage');
daqSession.Rate = 1000;
daqSession.DurationInSeconds = 10;
daqSession.startBackground();
```
**Logical Analysis:**
This code block creates a data acquisition session, adds an analog input channel, sets the sampling rate and duration, and then starts the background acquisition.
### 2.1.2 Data Preprocessing and Filtering
Data preprocessing and filtering are crucial for enhancing data quality and eliminating noise. The Control Systems Toolbox provides functions for performing these operations, such as `detrend`, `filter`, and `smoothdata`.
**Code Block:**
```matlab
data = detrend(data);
data = filter(b, a, data);
data = smoothdata(data, 'gaussian', 10);
```
**Logical Analysis:**
This code block uses the `detrend` function to remove trends from the data, the `filter` function to apply a digital filter, and the `smoothdata` function to smooth the data.
## 2.2 Data Analysis and Visualization
### 2.2.1 Time-Series Analysis and Modeling
The Control Systems Toolbox offers functions for performing time-series analysis and modeling, such as `autocorr`, `xcorr`, and `arx`. These functions can be used to identify patterns, trends, and correlations within the data.
**Code Block:**
```matlab
autocorr(data);
xcorr(data, data);
model = arx(data, [2 1 1]);
```
**Logical Analysis:**
This code block uses the `autocorr` function to calculate the autocorrelation of the data, the `xcorr` function to calculate the cross-correlation between data sets, and the `arx` function to fit an AutoRegressive with eXogenous inputs (ARX) model.
### 2.2.2 Data Visualization and Interactive Exploration
The Control Systems Toolbox provides functions for data visualization and interactive exploration, such as `plot`, `stem`, and `interactiveTimeSeries`. These functions can be used to create charts, graphs, and interactive interfaces for exploring data.
**Code Block:**
```matlab
plot(data);
stem(data);
interactiveTimeSeries(data);
```
**Logical Analysis:**
This code block uses the `plot` function to create a line graph of the data, the `stem` function to create a stem-and-leaf plot of the data, and the `interactiveTimeSeries` function to create an interactive time series graph.
# Practical Applications of the Control Systems Toolbox
### 3.1 System Modeling and Simulation
#### 3.1.1 Linear System Modeling and Simulation
**Linear System Modeling**
Linear systems can be represented using state-space models or transfer function models. A state-space model consists of state equations and output equations:
```
ẋ = Ax + Bu
y = Cx + Du
```
Where:
* x is the state vector
* u is the input vector
* y is the output vector
* A, B, C, D are system matrices
A transfer function model is represented by the transfer function between the input and output:
```
G(s) = Y(s)/U(s) = C(sI - A)^-1B + D
```
**Linear System Simulation**
The `lsim` function can be used for simulating linear systems. The `lsim` function requires system matrices `A`, `B`, `C`, `D`, and an input signal `u` as input parameters.
```
% System matrices
A = [0 1; -1 -2];
B = [0; 1];
C = [1 0];
D = 0;
% Input signal
t = 0:0.01:10;
u = sin(t);
% Simulation
[y, t, x] = lsim(A, B, C, D, u, t);
```
#### 3.1.2 Nonlinear System Modeling and Simulation
**No
0
0
相关推荐
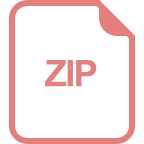
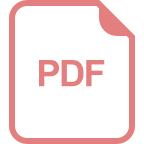
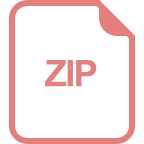





