MATLAB if Statement Performance Optimization: 5 Tips for Improving Code Efficiency
发布时间: 2024-09-13 18:04:11 阅读量: 38 订阅数: 27 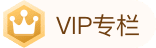
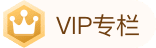
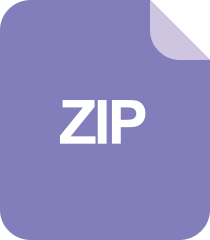
Bisection Method for unimodal function Optimization:Bisection Method for unimodal function optimization-matlab开发
# 1. Basic Concepts of MATLAB if Statements
A conditional statement in MATLAB, the if statement is used to execute or skip blocks of code based on given conditions. The syntax is as follows:
```
if condition
% Execute code block 1
else
% Execute code block 2
end
```
Here, `condition` is a logical expression that, if true, causes code block 1 to be executed; otherwise, code block 2 is executed. if statements can be nested to create complex conditional logic.
# 2. Tips for Optimizing if Statement Performance
### 2.1 Avoid Nested if Statements
#### 2.1.1 Use elseif and else
Nested if statements can degrade code readability and maintainability, and may also lead to performance issues. To avoid nested if statements, use elseif and else clauses.
**Example:**
```
% Nested if statement
if condition1
if condition2
% Code block 1
else
% Code block 2
end
else
% Code block 3
end
```
```
% Using elseif and else
if condition1
% Code block 1
elseif condition2
% Code block 2
else
% Code block 3
end
```
#### 2.1.2 Use switch-case Statements
When you need to execute different code blocks based on multiple conditions, switch-case statements can be used. These are more concise and efficient than nested if statements.
**Example:**
```
% Nested if statement
if condition1
if condition2
% Code block 1
elseif condition3
% Code block 2
else
% Code block 3
end
else
% Code block 4
end
```
```
% Using switch-case statements
switch condition1
case 1
% Code block 1
case 2
% Code block 2
case 3
% Code block 3
otherwise
% Code block 4
end
```
### 2.2 Vectorized Operations
#### 2.2.1 Avoid Loops
Loops can slow down code, especially when dealing with large datasets. To avoid loops, use vectorized operations. Vectorized operations apply loop operations to entire arrays or matrices, rather than to individual elements.
**Example:**
```
% Using a loop
for i = 1:length(x)
y(i) = x(i) + 1;
end
```
```
% Using vectorized operations
y = x + 1;
```
#### 2.2.2 Use Logical Indexing
Logical indexing allows you to select elements from an array or matrix based on a condition. Using logical indexing can avoid loops when filtering data.
**Example:**
```
% Using a loop
for i = 1:length(x)
if x(i) > 0
y(i) = x(i);
end
end
```
```
% Using logical indexing
y = x(x > 0);
```
### 2.3 Preallocate Memory
#### 2.3.1 Avoid Repeated Allocation
When memory needs to be dynamically allocated inside a loop, there is a performance overhead. To avoid repeated allocation, preallocate memory. Preallocation allocates sufficient space in one go to store the data generated in the loop.
**Example:**
```
% Avoiding repeated allocation
for i = 1:length(x)
y(i) = x(i) + 1;
end
```
```
% Preallocating memory
y = zeros(1, length(x));
for i = 1:length(x)
y(i) = x(i) + 1;
end
```
#### 2.3.2 Use Preallocation Functions
MATLAB provides preallocation functions like zeros, ones, and nan, which can be used to preallocate memory. Using these functions can improve code efficiency and readability.
**Example:**
```
% Using a preallocation function
y = zeros(1, length(x));
for i = 1:length(x)
y(i) = x(i) + 1;
end
```
# 3. Practical Applications of if Statements
### 3.1 Data Processing and Analysis
#### 3.1.1 Conditional Filtering and Selection
if statements are widely used in data processing and analysis to filter and select data based on specific conditions. For example, the following code uses an if statement to select elements greater than 50 from a dataset:
```matlab
data = [10, 20, 30, 40, 50, 60, 70, 80, 90, 100];
filtered_data = data(data > 50);
disp(filtered_data)
```
**Code logic analysis:**
* The `data` variable is an array containing 10 elements.
* `data > 50` creates a logical array where elements greater than 50 are true and others are false.
* `data(data > 50)` uses logical indexing to select elements from the `data` array that meet the condition.
* `disp(filtered_data)` displays the filtered data.
#### 3.1.2 Data Categorization and Grouping
if statements can also be used to categorize and group data. For instance, the following code uses an if statement to classify student grades into categories of excellent, good, average, pass, and fail:
```matlab
grades = [90, 80, 70, 60, 50, 40, 30, 20, 10];
categories = {'excellent', 'good', 'average', 'pass', 'fail'};
for i = 1:length(grades)
if grades(i) >= 90
category = categories{1};
elseif grades(i) >= 80
category = categories{2};
elseif grades(i) >= 70
category = categories{3};
elseif grades(i) >= 60
category = categories{4};
else
category = categories{5};
end
disp(['Grade: ', num2str(grades(i)), ', Category: ', category]);
end
```
**Code logic analysis:**
* The `grades` variable is an array containing student grades.
* The `categories` variable is a cell array containing category names.
* The `for` loop iterates through each grade in the `grades` array.
* The `if` statement uses nested conditions to determine the category of the grade.
* The `disp` statement displays the grade and its corresponding category.
### 3.2 Image Processing
#### 3.2.1 Image Segmentation and Enhancement
if statements are used in image processing to segment and enhance images based on specific conditions. For example, the following code sets red pixels to white in an image:
```matlab
image = imread('image.jpg');
red_channel = image(:,:,1);
for i = 1:size(red_channel, 1)
for j = 1:size(red_channel, 2)
if red_channel(i, j) > 128
red_channel(i, j) = 255;
end
end
end
enhanced_image = cat(3, red_channel, image(:,:,2), image(:,:,3));
imshow(enhanced_image)
```
**Code logic analysis:**
* The `image` variable is a 3D array containing image data.
* The `red_channel` variable extracts the red channel from the image.
* The nested `for` loops iterate over each pixel in the image.
* The `if` statement checks if the red value of each pixel is greater than 128. If it is, the pixel value is set to 255 (white).
* The `enhanced_image` variable recombines the modified red channel with the other channels.
* The `imshow` function displays the enhanced image.
#### 3.2.2 Feature Extraction and Pattern Recognition
if statements are also used in image processing to extract features and recognize patterns. For instance, the following code detects circles in an image:
```matlab
image = imread('image.jpg');
gray_image = rgb2gray(image);
binary_image = im2bw(gray_image, 0.5);
[centers, radii] = imfindcircles(binary_image, [10 50]);
for i = 1:length(centers)
if radii(i) > 20 && radii(i) < 30
viscircles(centers(i, :), radii(i), 'Color', 'r');
end
end
```
**Code logic analysis:**
* The `image` variable is a 3D array containing image data.
* The `gray_image` variable converts the image to grayscale.
* The `binary_image` variable converts the grayscale image to a binary image.
* The `imfindcircles` function detects circles in the image and returns their centers and radii.
* The nested `for` loop iterates over the detected circles.
* The `if` statement checks if the radius of the circle is between 20 and 30. If it is, the `viscircles` function is used to draw the circle on the image.
### 3.3 Signal Processing
#### 3.3.1 Signal Filtering and Noise Reduction
if statements are used in signal processing to filter and reduce noise based on specific conditions. For example, the following code filters out frequencies higher than 50 Hz from a signal:
```matlab
signal = load('signal.mat');
fs = 1000; % Sampling frequency
frequencies = linspace(0, fs/2, length(signal.data)/2);
fft_signal = fft(signal.data);
for i = 1:length(frequencies)
if frequencies(i) > 50
fft_signal(i) = 0;
end
end
filtered_signal = ifft(fft_signal);
```
**Code logic analysis:**
* The `signal` variable is a struct containing signal data.
* The `fs` variable is the sampling frequency.
* The `frequencies` variable is an array of signal frequencies.
* The `fft_signal` variable is the Fourier transform of the signal.
* The `for` loop iterates over the signal's frequencies.
* The `if` statement checks if the frequency is greater than 50 Hz. If it is, the corresponding Fourier transform coefficient is set to 0.
* The `filtered_signal` variable is the filtered signal.
# 4. Advanced Applications of if Statements
### 4.1 Dynamic Code Generation
#### 4.1.1 Using eval and feval Functions
MATLAB provides `eval` and `feval` functions that allow dynamically generating and executing code. These are particularly useful in the following scenarios:
***Code is dynamically generated:** When the code needs to change based on runtime conditions or user input.
***Improved code flexibility and reusability:** Allows code to be stored as strings or function handles and called upon as needed.
The `eval` function executes a string as MATLAB code. The `feval` function calls a function handle or a function specified by a string.
**Code block:**
```matlab
% Using the eval function to dynamically generate code
code_str = 'x = 2*x + 1;';
eval(code_str); % Execute dynamically generated code
% Using the feval function to call a function handle
func_handle = @(x) x^2 + 1;
result = feval(func_handle, 3); % Call function handle
```
**Logic analysis:**
* The `eval` function executes the `code_str` string as MATLAB code, updating the value of the `x` variable to 5.
* The `feval` function calls the `func_handle` function handle, passing 3 as a parameter and returning the result 10.
#### 4.1.2 Improving Code Flexibility and Reusability
Dynamic code generation allows code to be stored as strings or function handles and called upon as needed. This enhances code flexibility and reusability:
***Code reuse:** Store general code snippets as function handles or strings and call them multiple times as needed.
***Dynamic configuration:** Dynamically configure code based on runtime conditions or user input.
***Code generation:** Generate and save code as a file or execute it directly.
### 4.2 Parallel Programming
#### 4.2.1 Using parfor and spmd
MATLAB provides `parfor` and `spmd` functions for parallel programming. These are particularly useful in the following situations:
***Large-scale computations:** When computational load is high enough to benefit from parallel processing.
***Reducing execution time:** By distributing tasks across multiple processors, execution time can be reduced.
The `parfor` function executes for-loops in parallel. The `spmd` function creates multiple MATLAB workspaces, allowing code to be executed in parallel in each workspace.
**Code block:**
```matlab
% Using parfor to execute for-loops in parallel
parfor i = 1:10000
% Calculate the square of the i-th element
result(i) = i^2;
end
% Using spmd to create parallel MATLAB workspaces
spmd
% Calculate the cube of the i-th element in each workspace
result(labindex) = labindex^3;
end
```
**Logic analysis:**
* The `parfor` function executes the for-loop in parallel on multiple processors.
* The `spmd` function creates 12 parallel MATLAB workspaces (equal to the number of processors). Each workspace calculates the cube of the `labindex` element.
#### 4.2.2 Improving Computational Efficiency and Reducing Execution Time
Parallel programming can improve computational efficiency and reduce execution time by distributing tasks across multiple processors. This is particularly useful in the following scenarios:
***CPU-intensive computations:** When computations require significant CPU resources.
***Handling large datasets:** When datasets are too large to be efficiently processed on a single processor.
***Real-time applications:** When rapid response times are necessary, such as in control systems or data stream analysis.
# 5. Best Practices for if Statement Performance Optimization
### 5.1 Code Readability and Maintainability
#### 5.1.1 Use Clear Naming Conventions
* Use meaningful and descriptive variable and function names.
* Avoid using abbreviations or ambiguous names.
* Follow a consistent naming convention, such as camelCase or underscore separation.
#### 5.1.2 Add Comments and Documentation
* Add clear comments to the code explaining its purpose and logic.
* Use document strings (e.g., MATLAB's `help` function) to provide more detailed information.
* Write self-documenting code so other developers can easily understand and maintain it.
### 5.2 Performance Analysis and Tuning
#### 5.2.1 Use the Profiler Function
* Use MATLAB's `profiler` function to analyze code performance.
* Identify functions and code segments that take the most time.
* Determine performance bottlenecks and take measures to optimize.
#### 5.2.2 Identify and Eliminate Performance Bottlenecks
* Avoid unnecessary loops and repeated calculations.
* Use vectorized operations to improve efficiency.
* Preallocate memory to reduce allocation and deallocation operations.
* Consider using parallel programming techniques to increase computation speed.
**Code Example:**
```matlab
% Use clear naming conventions
function [mean_value, std_dev] = calculate_statistics(data)
% Add comments and documentation
% Calculate the mean and standard deviation of data
mean_value = mean(data);
std_dev = std(data);
```
**Performance Analysis Example:**
```matlab
% Use the profiler function
profile on;
calculate_statistics(large_data_array);
profile off;
profile viewer;
```
By following these best practices, you can write if statements that are not only performance optimized but also highly readable and maintainable. This will help improve the overall efficiency and reliability of your MATLAB applications.
0
0
相关推荐







