【Basic】Detailed Explanation of MATLAB Toolbox: Communications Toolbox
发布时间: 2024-09-14 04:04:26 阅读量: 67 订阅数: 39 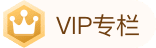
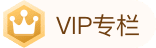
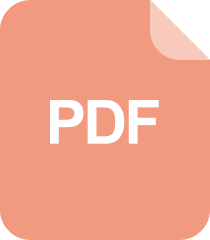
MATLAB课件:ch4_functions_and_scopes.pdf
# 1. Introduction to MATLAB Communications Toolbox
The MATLAB Communications Toolbox is a powerful toolkit designed for the design, simulation, and analysis of communication systems. It offers a wide array of functions and tools that encompass all aspects of communication system development, from signal generation and analysis to modulation, demodulation, and channel coding.
The Communications Toolbox is adept at simulating and designing various communication systems, including wireless, wired, and optical fiber networks. It also provides a range of pre-built modules and examples for a quick and easy start.
# 2. Fundamental Theories of Communication Systems
### 2.1 Communication System Model
A communication system is one that transmits information from one point to another. It consists of the following main components:
- **Information Source:** Produces the information to be transmitted.
- **Transmitter:** Converts the information into a signal suitable for transmission.
- **Channel:** The medium through which the signal is transmitted.
- **Receiver:** Restores the information from the signal.
- **Destination:** The final point where the information is received.
The communication system model can be represented as:
```
Information Source -> Transmitter -> Channel -> Receiver -> Destination
```
### 2.2 Modulation and Demodulation Techniques
Modulation is the process of converting an information signal into one that is suitable for transmission t***
***mon modulation techniques include:
- **Amplitude Modulation (AM):** Modifies the amplitude of the carrier signal to represent information.
- **Frequency Modulation (FM):** Modifies the frequency of the carrier signal to represent information.
- **Phase Modulation (PM):** Modifies the phase of the carrier signal to represent information.
Demodulation techniques correspond to modulation techniques, such as AM demodulators, FM demodulators, and PM demodulators.
### 2.3 Channel Coding and Decoding
Channel coding involves adding redundant bits to the information before transmission to improve the reliability of information recovery by the receiver in the presence of channel no***
***mon channel coding techniques include:
- **Convolutional Coding:** Converts the information bit stream into convolutional codewords.
- **Reed-Solomon Coding:** Converts the information bit stream into Reed-Solomon codewords.
Decoding techniques correspond to coding techniques, such as convolutional decoders and Reed-Solomon decoders.
**Code Block:**
```matlab
% Convolutional Encoding
encodedBits = convEncode(messageBits, generator, trellis);
% Convolutional Decoding
decodedBits = convDecode(receivedBits, generator, trellis);
```
**Logical Analysis:**
* The `convEncode` function uses the specified generator polynomial and trellis structure to convolutionally encode the information bit stream `messageBits`, generating the encoded bit stream `encodedBits`.
* The `convDecode` function uses the same generator polynomial and trellis structure to convolutionally decode the received bit stream `receivedBits`, generating the decoded bit stream `decodedBits`.
# 3. Practical Application of Communications Toolbox
### 3.1 Signal Generation and Analysis
The MATLAB Communications Toolbox provides a suite of functions for generating and analyzing various types of signals. These functions can be used to create test signals for communication system simulation and modeling.
**Signal Generation**
***comm.SignalSource:** Generates various signals such as sine waves, square waves, pulses, and noise.
***comm.ChirpGenerator:** Generates linear frequency-modulated (LFM) signals.
***comm.OFDMModulator:** Generates orthogonal frequency division multiplexing (OFDM) signals.
**Signal Analysis**
***comm.SpectrumAnalyzer:** Analyzes the frequency spectrum of a signal.
***comm.TimeScope:** Visualizes the time-domain waveform of a signal.
***comm.PhaseDetector:** Measures the phase shift of a signal.
### 3.2 Implementation of Modulation and Demodulation Algorithms
The Communications Toolbox provides functions for implementing various modulation and demodulation algorithms. These functions can be used to build custom communication systems or analyze existing systems.
**Modulation**
***comm.PSKModulator:** Modulates the signal using phase shift keying (PSK).
***comm.QAMModulator:** Modulates the signal using quadrature amplitude modulation (QAM).
***comm.OFDMModulator:** Modulates the signal using orthogonal frequency division multiplexing (OFDM).
**Demodulation**
***comm.PSKDemodulator:** Demodulates signals modulated using phase shift keying (PSK).
***comm.QAMDemodulator:** Demodulates signals modulated using quadrature amplitude modulation (QAM).
***comm.OFDMDemodulator:** Demodulates signals modulated using orthogonal frequency division multiplexing (OFDM).
### 3.3 Channel Simulation and Modeling
The Communications Toolbox includes functions for simulating and modeling various channels. These functions can be used to evaluate the performance of communication systems and optimize their design.
**Channel Simulation**
***comm.AWGNChannel:** Simulates a channel with additive white Gaussian noise (AWGN).
***comm.RayleighChan
0
0
相关推荐
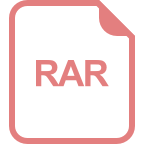
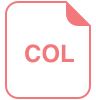
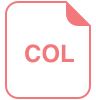
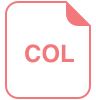
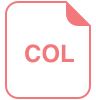
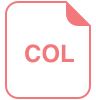
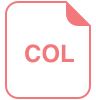
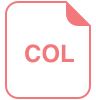