【Basic】Detailed Explanation of MATLAB Toolboxes: Image Processing Toolbox
发布时间: 2024-09-14 03:32:51 阅读量: 38 订阅数: 45 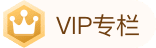
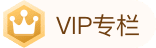
# 2.1 Image Representation and Data Types
### 2.1.1 Pixels and Color Spaces in Images
An image is fundamentally composed of pixels, where each pixel represents the color information at a specific location within the image. Pixels are typically represented by three values: red, green, and blue (RGB), known as color channels. The values for these channels range from 0 to 255, where 0 signifies black and 255 signifies white.
The color space of an image defines how colors are represented within the image. The most common color space is RGB, which uses three channels to represent colors. Other color spaces include grayscale (which uses a single channel to represent brightness), CMYK (used for printing), and HSV (used for image processing).
# 2. Fundamental Theories of Image Processing
### 2.1 Image Representation and Data Types
#### 2.1.1 Pixels and Color Spaces in Images
An image consists of pixels, each representing the color at a particular location within the image. Pixel values are often represented numerically, indicat***
***mon color spaces include:
- **RGB (Red, Green, Blue)**: Represents colors as a combination of the three primary colors.
- **HSV (Hue, Saturation, Value)**: Represents colors in terms of hue, saturation, and brightness.
- **CMYK (Cyan, Magenta, Yellow, Key)**: A subtractive color model used for printing.
#### ***
***mon image data types include:
- **uint8**: An 8-bit unsigned integer with a range of [0, 255], suitable for storing grayscale images.
- **uint16**: A 16-bit unsigned integer with a range of [0, 65535], suitable for storing color images.
- **double**: A 64-bit floating-point number with a range of [-Inf, Inf], suitable for storing high-precision images.
When selecting an image data type, factors such as image accuracy, storage space, and processing speed must be considered.
### 2.2 Image Processing Algorithms
Image processing algorithms are used to manipulate and analyze images to extract information or enhance visual effects. Image processing algorithms can be categorized into several types:
#### 2.2.1 Image Enhanc***
***mon image enhancement algorithms include:
- **Contrast Enhancement**: Adjusts the contrast of the image to make it clearer.
- **Histogram Equalization**: Adjusts the histogram of the image to provide a more uniform distribution of brightness.
- **Sharpening**: Enhances edges and details within the image.
#### 2.2.2 Image Segmentation
Image segmentation algorithms divide an image into different regions, ***mon image segmentation algorithms include:
- **Thresholding Segmentation**: Divides the image into different regions based on pixel intensity or color.
- **Region Growing Segmentation**: Starts from seed points and groups adjacent similar pixels into the same region.
- **Clustering Segmentation**: Clusters pixels within the image into different groups, with each group representing an object within the image.
#### 2.2.3 Image Feature Extraction
Image feature extraction algorithms extract useful features from images, which can be used for object recognition, classification, ***mon image feature extraction algorithms include:
- **Edge Detection**: Detects edges and contours within the image.
- **Feature Point Detection**: Detects key points within the image, such as corners and blobs.
- **Texture Analysis**: Analyzes the texture patterns within the image to extract texture features.
# 3.1 Image Reading and Display
#### 3.1.1 Use of imread Function
The `imread` function is used to read image files and convert them into MATLAB arrays. The syntax is as follows:
```
I = imread(filename)
```
Where:
- `I`: The output image array, which can be of type `uint8` or `double`, depending on the type of the input image.
- `filename`: The full path and filename of the image file, including the extension.
**Code Block:**
```matlab
% Read the image file
I = imread('image.jpg');
% Display the image
imshow(I);
```
**Logical Analysis:**
- `imread('image.jpg')` reads the image file named "image.jpg" and converts it into a MATLAB array `I`.
- `imshow(I)` displays the image array `I`.
#### 3.1.2 Use of imshow Function
The `imshow` function is used to display image arrays. The syntax is as follows:
```
imshow(I)
```
Where:
- `I`: The image array to be displayed.
**Code Block:**
```matlab
% Read the image file
I = imread('image.jpg');
% Display the image
imshow(I);
```
**Logical Analysis:**
- `imread('image.jpg')` reads the image file named "image.jpg" and converts it into a MATLAB array `I`.
- `imshow(I)` displays the image array `I`.
**Parameter Explanation:**
- `'InitialMagnification'`: Specifies the initial magnification level of the image. The default value is 1.
- `'Border'`: Specifies the color of the border around the image. The default value is 'tight', which means the image is displayed close to the border.
- `'DisplayRange'`: Specifies the display range for the image, used to adjust the contrast. The default value is 'auto', which means the contrast is automatically adjusted.
# 4.1 Image Feature Extraction and Analysis
Image feature extraction is a crucial step in image processing, capable of extracting important information from images, providing a foundation for subsequent image analysis and recognition. The Image Processing Toolbox offers a wealth of image feature extraction algorithms, including edge detection, feature point detection, and texture analysis.
### 4.1.1 Edge Detection
Edge detection is a vital technique in image processing for extracting the contours and boundaries of objects within an image. The Image Processing Toolbox provides various edge detection algorithms, including:
- **Sobel Operator**: Uses a first-order differential operator to detect edges in an image.
- **Canny Operator**: Uses a multi-level edge detection algorithm that effectively detects edges in an image while suppressing noise.
- **Prewitt Operator**: Similar to the Sobel operator but uses different convolution kernels.
```matlab
% Load the image
I = imread('image.jpg');
% Perform edge detection using the Sobel operator
edges = edge(I, 'Sobel');
% Display the edge detection result
figure;
imshow(edges);
title('Sobel Edge Detection');
```
### 4.1.2 Feature Point Detection
Feature point detection can identify points with significant changes within an image, which often correspond to key features in the image. The Image Processing Toolbox offers various feature point detection algorithms, including:
- **Harris Corner Detection**: Detects points with high curvature in an image, which typically correspond to corners in the image.
- **SIFT (Scale-Invariant Feature Transform)**: Detects feature points that are scale-invariant and rotation-invariant in an image.
- **SURF (Speeded-Up Robust Features)**: Similar to SIFT but faster in computation.
```matlab
% Load the image
I = imread('image.jpg');
% Use the Harris corner detection algorithm
corners = detectHarrisFeatures(I);
% Display the corner detection result
figure;
imshow(I);
hold on;
plot(corners.Location(:,1), corners.Location(:,2), 'ro');
title('Harris Corner Detection');
```
### 4.1.3 Texture Analysis
Texture analysis can extract features from the texture within an image, which can be used for tasks such as image classification and object detection. The Image Processing Toolbox provides various texture analysis algorithms, including:
- **Gray-Level Co-occurrence Matrix (GLCM)**: Computes statistical features of pixel pairs in an image based on their distance and direction.
- **Local Binary Pattern (LBP)**: Computes the binary pattern of pixels around each pixel in an image.
- **Scale-Invariant Feature Transform (SIFT)**: Can also be used for texture analysis, as it can extract texture features that are scale-invariant.
```matlab
% Load the image
I = imread('image.jpg');
% Compute the gray-level co-occurrence matrix
glcm = graycomatrix(I);
% Compute texture features
stats = graycoprops(glcm, {'Contrast', 'Correlation', 'Energy', 'Homogeneity'});
% Display texture features
disp(stats);
```
# 5. Integration of Image Processing Toolbox with Other Tools
### 5.1 Integration of MATLAB and Python
MATLAB and Python are two programming languages widely used for scientific computation and data analysis. Integrating these two can leverage their respective strengths, enabling more powerful image processing capabilities.
#### 5.1.1 Python Calls MATLAB Functions
Python can call MATLAB functions through the `matlab.engine` module. This module provides an interface that allows Python scripts to interact with the MATLAB engine.
```python
import matlab.engine
# Start a MATLAB engine
eng = matlab.engine.start_matlab()
# Call a MATLAB function
result = eng.my_matlab_function(1, 2)
# Stop the MATLAB engine
eng.quit()
```
#### 5.1.2 MATLAB Calls Python Libraries
MATLAB can call Python libraries via the `py.import` function. This function returns a Python module object, through which Python functions and classes can be accessed.
```matlab
% Import a Python library
py_module = py.importlib.import_module('my_python_module');
% Call a Python function
result = py_module.my_python_function(1, 2);
```
### 5.2 Integration of Image Processing Toolbox with Deep Learning Frameworks
Deep learning frameworks such as TensorFlow and PyTorch provide powerful features for image processing. Integrating the Image Processing Toolbox with these frameworks can enable more complex and accurate image processing tasks.
#### 5.2.1 Combining TensorFlow and Image Processing Toolbox
TensorFlow is an open-source framework for machine learning and deep learning. It provides various modules for image processing, including image preprocessing, feature extraction, and classification.
```matlab
% Import TensorFlow
import tensorflow as tf
% Load an image using Image Processing Toolbox
image = imread('image.jpg');
% Convert the image to a TensorFlow tensor
image_tensor = tf.convert_to_tensor(image)
% Process the image using a TensorFlow model
processed_image = model(image_tensor)
```
#### 5.2.2 Combining PyTorch and Image Processing Toolbox
PyTorch is an open-source framework for deep learning. It provides modules for image processing, including image loading, data augmentation, and neural network models.
```python
import torch
# Load an image using Image Processing Toolbox
image = imread('image.jpg')
# Convert the image to a PyTorch tensor
image_tensor = torch.from_numpy(image)
# Process the image using a PyTorch model
processed_image = model(image_tensor)
```
# 6. Image Processing Toolbox Application Cases
### 6.1 Medical Image Processing
#### 6.1.1 Medical Image Segmentation
**Purpose:** To separate different tissues or organs within medical images into distinct areas for further analysis and diagnosis.
**Methods:**
1. **Manual Segmentation:** Manually outline the boundaries of the area of interest using a mouse or stylus.
2. **Semi-automatic Segmentation:** Use algorithms to pre-segment the image, then manually adjust the segmentation results.
3. **Fully Automatic Segmentation:** Automatically segment the image using machine learning or deep learning algorithms.
**Code Example:**
```matlab
% Load a medical image
I = imread('medical_image.jpg');
% Use Otsu's thresholding to segment the image
segmentedImage = im2bw(I, graythresh(I));
% Display the segmentation result
imshow(segmentedImage);
```
#### 6.1.2 Medical Image Enhancement
**Purpose:** To improve the contrast and clarity of medical images for more accurate diagnosis.
**Methods:**
1. **Histogram Equalization:** Adjust the image histogram to enhance contrast.
2. **Adaptive Histogram Equalization:** Apply local histogram equalization to different regions of the image.
3. **Sharpening:** Use filters to enhance edges and details within the image.
**Code Example:**
```matlab
% Load a medical image
I = imread('medical_image.jpg');
% Use adaptive histogram equalization to enhance the image
enhancedImage = adapthisteq(I);
% Display the enhancement result
imshow(enhancedImage);
```
### 6.2 Remote Sensing Image Processing
#### 6.2.1 Remote Sensing Image Classification
**Purpose:** To classify pixels within remote sensing images into different land cover types, such as vegetation, water bodies, and buildings.
**Methods:**
1. **Supervised Classification:** Train a classifier using known land cover types as training data.
2. **Unsupervised Classification:** Use clustering algorithms to group pixels into different categories without training data.
**Code Example:**
```matlab
% Load a remote sensing image
I = imread('remote_sensing_image.jpg');
% Perform supervised classification using a Support Vector Machine (SVM)
classifier = fitcsvm(features, labels);
classifiedImage = predict(classifier, features);
% Display the classification result
imshow(classifiedImage);
```
#### 6.2.2 Remote Sensing Image Object Detection
**Purpose:** To detect and locate specific objects within remote sensing images, such as vehicles, buildings, or ships.
**Methods:**
1. **Sliding Window:** Slide a window across the image and classify the pixels within the window using a classifier.
2. **Region-based Convolutional Neural Networks (R-CNN):** Use deep learning algorithms to generate candidate regions and then classify each region.
3. **You Only Look Once (YOLO):** Use a single convolutional neural network to detect and locate objects within the image.
**Code Example:**
```matlab
% Load a remote sensing image
I = imread('remote_sensing_image.jpg');
% Perform object detection using YOLOv3
net = yolov3('weights', 'yolov3.weights');
[bboxes, scores, labels] = detect(net, I);
% Display the detection results
imshow(I);
hold on;
for i = 1:length(bboxes)
rectangle('Position', bboxes(i, :), 'EdgeColor', 'r', 'LineWidth', 2);
end
hold off;
```
0
0
相关推荐








