MATLAB Toolbox Detailed Explanation: Statistics and Machine Learning Toolbox
发布时间: 2024-09-14 03:34:01 阅读量: 18 订阅数: 22 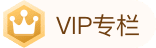
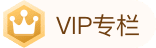
# Introduction to the MATLAB Statistics and Machine Learning Toolbox
The MATLAB Statistics and Machine Learning Toolbox is a powerful suite of functions and tools for statistical analysis and machine learning, designed to provide MATLAB users with extensive capabilities for data preprocessing, statistical modeling, and the development and deployment of machine learning algorithms. This toolbox is essential for data scientists, researchers, and engineers who need to harness MATLAB's robust computational power to tackle complex data analysis and machine learning challenges.
# Theoretical Foundations of the Statistics and Machine Learning Toolbox
### Statistical Fundamentals
#### Probability Theory
Probability theory is the foundation of statistics; it studies the likelihood of random events occu***mon probability distributions include the normal, binomial, and Poisson distributions.
```matlab
% Generating normal distribution data
data = normrnd(0, 1, 1000);
% Plotting a histogram of the normal distribution
histogram(data);
xlabel('Data Value');
ylabel('Frequency');
title('Normal Distribution Histogram');
% Calculating the mean and standard deviation of the normal distribution
mean_data = mean(data);
std_data = std(data);
% Printing the mean and standard deviation
fprintf('Mean: %.2f\n', mean_data);
fprintf('Standard Deviation: %.2f\n', std_data);
```
#### Statistical I***
***mon methods of statistical inference include hypothesis testing and confidence interval estimation.
```matlab
% Hypothesis testing: comparing the means of two normal distributions
[h, p] = ttest2(data1, data2);
% If p < 0.05, reject the null hypothesis, indicating that the means of the two distributions are different
if p < 0.05
fprintf('Reject null hypothesis: The means of the two distributions are different.\n');
else
fprintf('Fail to reject null hypothesis: The means of the two distributions are not different.\n');
end
% Confidence interval estimation: estimating the mean of a normal distribution
[mu, sigma] = normfit(data);
ci = normconfint(0.95, mu, sigma);
% Printing the confidence interval
fprintf('95%% Confidence Interval: [%.2f, %.2f]\n', ci(1), ci(2));
```
### Machine Learning Fundamentals
#### Supervised Learning
Supervised ***mon supervised learning algorithms include linear regression, logistic regression, and support vector machines.
```matlab
% Linear regression: predicting house prices
data = load('house_prices.mat');
% Feature variable: area
X = data.area;
% Label variable: house price
y = data.price;
% Training a linear regression model
model = fitlm(X, y);
% Predicting the house price for a new area
new_area = 2000;
predicted_price = predict(model, new_area);
% Printing the predicted house price
fprintf('Predicted Price for Area = 2000: %.2f\n', predicted_price);
```
#### Unsupervised Learning
Unsupervised learn***mon unsupervised learning algorithms include clustering, dimensionality reduction, and anomaly detection.
```matlab
% Clustering: grouping customers into different segments
data = load('customer_data.mat');
% Feature variables: age, income, expenditure
X = data.features;
% Training a K-Means clustering model
model = kmeans(X, 3);
% Predicting the segment for a new customer
new_customer = [30, 50000, 20000];
predicted_cluster = predict(model, new_customer);
% Printing the predicted segment
fprintf('Predicted Segment for New Customer: %d\n', predicted_cluster);
```
# Data Preprocessing
Data preprocessing is a crucial step in the machine learning workflow, as it can enhance the accuracy and efficiency of models. The Statistics and Machine Learning Toolbox offers a broad range of data preprocessing functionalities, including data cleaning and transformation.
#### Data Cleaning
Data cleaning involves identifying and addressing errors, missing values, and outliers within the data. The data cleaning functions provided in the Toolbox include:
- `findmissing()`: Identifies the locations of missing values in a dataset.
- `ismissing()`: Checks if a specific data point is missing.
- `replacemissing()`: Replaces missing values with a specified value, such as the mean or median.
- `outliers()`: Identifies potential outliers in a dataset.
- `removeoutliers()`: Removes identified outliers.
```matlab
% Importing data
data = importdata('data.csv');
% Finding missing values
missing_values = findmissing(data);
% Replacing missing values with the mean
data(missing_values) = mean(data, 1);
% Identifying outliers
outliers = outliers(data);
% Removing outliers
data(outliers, :) = [];
```
#### Data Transformation
Data transformation involves converting data from one format to another to better suit modeling purposes. The data transformation functions in the Toolbox include:
- `normalize()`: Normalizes data to a range between 0 and 1.
- `standardize()`: Standardizes data to have a mean of 0 and a standard deviation of 1.
- `pca()`: Performs Principal Component Analysis (PCA) to reduce data dimensionality.
- `lda()`: Performs Linear Discriminant Analysis (LDA) to project data into a subspace that best separates different classes.
```matlab
% Normalizing data
normalized_data = normalize(data);
% Standardizing data
standardized_data = standardize(data);
% Executing PCA
[coeff, score, latent] = pca(data);
% Executing LDA
[lda_coeff, lda_score] = lda(data, labels);
```
# Advanced Applications of the Statistics and Machine Learning Toolbox
### Time Series Analysis
#### Features of Time Series Data
Time series data is a sequence of observations collected over time. It possesses the following characteristics:
- **Trend**: The long-term pattern of data values gradually increasing or decreasing.
- **Seasonality**: The pattern of data values repeating at specific time intervals, such as daily, weekly, or annually.
- **Cyclicity**: The pattern of data values that repeat over longer intervals, typically longer than seasonality.
- **Randomness**: Variations in data values that cannot be explained by trend, seasonality, or cyclicity.
#### Time Series Models
The MATLAB Statistics and Machine Learning Toolbox provides various time series models, including:
- **Autoregressive Moving Average (ARMA) model**: Combines autoregressive (AR) and moving average (MA) models to capture trends and randomness in the data.
- **Autoregressive Integrated Moving Average (ARIMA) model**: An extension of the ARMA model that includes differencing operations to handle non-stationary data.
- **Exponential smoothing models**: Used for forecasting data with exponential decay trends.
- **State space models**: For handling time series data with underlying state variables.
**Code Block:**
```matlab
% Importing time series data
data = load('timeseries_data.mat');
data = data.timeseries_data;
% Creating an ARIMA model
model = arima(data, [1, 1, 1]);
% Predicting future values
forecast = forecast(model, 10);
% Plotting actual data and predicted values
figure;
plot(data, 'b', 'LineWidth', 2);
hold on;
plot(forecast, 'r--', 'LineWidth', 2);
legend('Actual Data', 'Predicted Data');
xlabel('Time');
ylabel('Value');
title('Time Series Prediction');
```
**Logical Analysis:**
- The `arima` function creates an ARIMA model with the order specified by `[1, 1, 1]`.
- The `forecast` function predicts the next 10 values using the model.
- Plotting code visualizes the actual data and predictions for comparison.
### Natural Language Processing
#### Text Preprocessing
Text preprocessing is a key step in natural language processing, involving tasks such as:
- **Tokenization**: Breaking text into words or phrases.
- **Stemming**: Reducing words to their base or root form.
- **Removing stop words**: Eliminating common, non-informative words like "the", "and", "of".
- **Normalization**: Converting text to lowercase, removing punctuation, etc.
#### Text Classification
The MATLAB Statistics and Machine Learning Toolbox provides algorithms for text classification, including:
- **Naive Bayes classifier**: A simple classifier based on Bayes' theorem, which assumes feature independence.
- **Support Vector Machine (SVM)**: Uses a hyperplane to separate data points into different categories.
- **Decision tree**: Recursively assigns data points to categories through a series of rules.
**Code Block:**
```matlab
% Importing text data
data = readtable('text_data.csv');
% Text preprocessing
data.text = lower(data.text);
data.text = removePunctuation(data.text);
data.text = removeStopWords(data.text);
% Creating a text classifier
classifier = fitcnb(data.text, data.category);
% Predicting the category of new text
new_text = 'This is a new text to classify.';
predicted_category = predict(classifier, new_text);
```
**Logical Analysis:**
- The `readtable` function imports text data from a CSV file.
- The text preprocessing code performs tokenization, punctuation removal, and stop word removal.
- The `fitcnb` function creates a Naive Bayes classifier.
- The `predict` function classifies the new text using the classifier.
### Image Processing
#### Image Enhancement
Image enhancement techniques are used to improve the visual quality of images, including:
- **Contrast enhancement**: Adjusting the brightness range of pixels in an image.
- **Histogram equalization**: Redistributing the brightness values of pixels in an image to enhance contrast.
- **Sharpening**: Increasing the clarity of edges in an image.
#### Image Segmentation
Image segmentation divides an image into regions with different characteristics, including:
- **Thresholding segmentation**: Segregating an image into a binary image based on pixel brightness.
- **Region growing segmentation**: Grouping similar pixels into a region starting from a seed point.
- **Edge detection**: Identifying edges in an image where there are changes in brightness.
**Code Block:**
```matlab
% Importing an image
image = imread('image.jpg');
% Image enhancement
enhanced_image = imadjust(image, [0.2, 0.8], []);
% Image segmentation
segmented_image = im2bw(enhanced_image, 0.5);
% Displaying images
figure;
subplot(1, 3, 1);
imshow(image);
title('Original Image');
subplot(1, 3, 2);
imshow(enhanced_image);
title('Enhanced Image');
subplot(1, 3, 3);
imshow(segmented_image);
title('Segmented Image');
```
**Logical Analysis:**
- The `imread` function imports an image.
- The `imadjust` function enhances the image's contrast.
- The `im2bw` function converts the image into a binary image using a threshold of 0.5 for segmentation.
- The plotting code displays the original image, the enhanced image, and the segmented image.
# Model Optimization
### Hyperparameter Tuning
Hyperparameter tuning is a key step in optimizing the performance of machine learning models. Hyperparameters are parameters set during the model training process that are not learned from the data, such as learning rate, regularization parameters, etc.
**Grid Search for Hyperparameter Tuning**
Grid search is a widely used method for hyperparameter tuning. It involves systematically traversing a predefined grid of hyperparameter values and selecting the combination that yields the best performance.
```matlab
% Defining the hyperparameter grid
param_grid = {
'LearningRate', [0.01, 0.001, 0.0001],
'Regularization', [0.1, 0.01, 0.001]
};
% Performing grid search
[best_params, best_score] = gridSearch(model, param_grid, data);
```
### Regularization
Regularization is a technique used to prevent machine learning models from overfitting. Overfitting occurs when a model performs well on the training data but poorly on new data.
**L1 Regularization**
L1 regularization adds a term to the loss function that penalizes the absolute value of model weights, encouraging a sparse solution.
```matlab
% L1 Regularization
model = trainModel(data, 'L1Regularization', 0.1);
```
**L2 Regularization**
L2 regularization adds a term to the loss function that penalizes the square of model weights, encouraging a smooth solution.
```matlab
% L2 Regularization
model = trainModel(data, 'L2Regularization', 0.1);
```
0
0
相关推荐
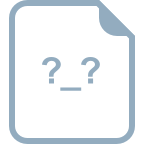
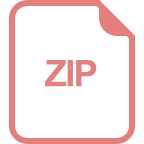
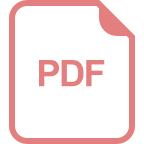





