【Advanced Level】Design and Implementation of Distributed Crawler Architecture
发布时间: 2024-09-15 12:21:09 阅读量: 17 订阅数: 32 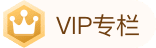
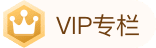
# [Advanced] Design and Implementation of Distributed Crawler Architecture
## 1. Overview of Distributed Crawler
A distributed crawler is a distributed computing system that assigns crawling tasks to multiple distributed nodes for concurrent execution, ***pared with traditional centralized crawlers, distributed crawlers have the following advantages:
- **High concurrency:** Distributed crawlers can use multiple nodes to concurrently fetch, significantly improving crawling efficiency.
- **High reliability:** Nodes in a distributed crawler can back each other up. If a node fails, other nodes can continue executing the crawling tasks, ensuring stable operation.
- **Scalability:** Distributed crawlers can easily scale the crawling size by increasing or decreasing the number of nodes to meet the needs of different scales.
## 2. Design of Distributed Crawler Architecture
### 2.1 Components of a Distributed Crawler
A distributed crawler system consists of several collaborative components, each responsible for specific functions. The main modules include:
- **Crawler node:** Responsible for fetching web content from target websites and extracting the required information.
- **Scheduling center:** Responsible for distributing crawling tasks, monitoring the crawler status, and coordinating the operation of the entire crawler system.
- **Storage center:** Responsible for storing the fetched web content and extracted information, and providing query and analysis functions.
### 2.2 Communication Mechanism of Distribute***
***mon communication mechanisms include:
- **Message queues:** Used for asynchronous message passing between components, achieving loose coupling and scalability.
- **RPC framework:** Used for remote procedure calls between components, achieving synchronous communication and service discovery.
### 2.3 Load Balancing of Distributed Crawler
To improve the efficiency and reliability of the crawler system, it is necessary to perform load balancing on crawling tasks, ***mon load balancing algorithms include:
- **Hash-based load balancing:** Distributes tasks based on hash values to ensure uniform distribution.
- **Weight-based load balancing:** Assigns different weights to nodes based on their performance and resources, prioritizing task distribution to nodes with higher weights.
**Code block: Hash-based Load Balancing**
```python
import hashlib
def hash_based_load_balancing(task, nodes):
"""
Hash-based load balancing algorithm.
Parameters:
task: Task to be assigned.
nodes: List of crawler nodes.
Returns:
Crawler node assigned to the task.
"""
# Calculate hash value of the task
task_hash = hashlib.md5(task.encode()).hexdigest()
# Select crawler node based on hash value
node_index = int(task_hash, 16) % len(nodes)
return nodes[node_index]
```
**Logical Analysis:**
This code block implements a hash-based load balancing algorithm. It first calculates the hash value of the task to be assigned. Then, it selects the crawler node based on the hash value. The hash value is converted to an integer, and the modulo operation is performed on the length of the crawler node list to determine the index of the crawler node to which the task is assigned.
**Parameter Description:**
- `task`: Task to be assigned.
- `nodes`: List of crawler nodes.
**Mermaid Format Flowchart: Weight-based Load Balancing**
```mermaid
sequenceDiagram
participant Dispatcher
participant Node1
participant Node2
participant Node3
Dispatcher->Node1: Assign task with weight 1
Dispatcher->Node2: Assign task with weight 2
Dispatcher->Node3: Assign task with weight 3
```
**Logical Analysis:**
This flowchart illustrates a weight-based load balancing algorithm. The dispatcher assigns tasks based on the weights of the crawler nodes. Nodes with higher weights will receive more tasks.
**Parameter Description:**
- `Dispatcher`: Dispatcher.
- `Node1`, `Node2`, `Node3`: Crawler nodes.
- `weight`: Weight of the crawler node.
## 3. Implementation of Distributed Crawler
### 3.1 Implementation of Crawler Nodes
#### 3.1.1 Acquisition and Execution of Crawler Tasks
A crawler node is a component in a distributed crawler system responsible for fetching web content. It obtains crawling tasks from the scheduling center, executes them, parses the web content, and extracts the required data.
**Code Block:**
```python
import requests
from bs4 import BeautifulSoup
def get_html(url):
"""
Fetches the HTML content of a webpage.
Args:
url: Webpage URL.
Returns:
HTML content of the webpage.
"""
response = requests.get(url)
if response.status_code == 200:
return response.text
else:
return None
def parse_html(html):
"""
Parses the HTML content of a webpage and extracts the required data.
Args:
html: HTML content of the webpage.
Returns:
Extracted data.
"""
soup = BeautifulSoup(html, 'html.parser')
data = []
for item in soup.find_all('div', class_='item'):
title = item.find('h2').text
link = item.find('a')['href']
data.append({
'title': title,
'link': link
})
return data
def execute_task(task):
"""
Executes a crawling task.
Args:
task: Crawling task.
"""
url = task['url']
html = get_html(url)
if html:
data = parse_html(html)
# Store extracted data into a database or other storage medium
else:
# Handle cases where fetching fails, such as retrying or logging errors
```
**Code Logic Analysis:**
* The `get_html` function uses the `requests` library to fetch HTML content.
* The `parse_html` function uses `BeautifulSoup` to parse HTML content and extract data.
* The `execute_task` function executes the crawling task, including fetching HTML content, parsing HTML content, and extracting data.
#### 3.1.2 Parsing and Extraction of Webpage Content
When a crawler node parses webpage content, ***mon parsing tools include regular expressions, HTML parsing libraries (such as BeautifulSoup), and XPath.
**Code Block:**
```python
import re
def extract_phone_numbers(html):
"""
Extracts phone numbers from HTML content.
Args:
html: Webpage HTML content.
Returns:
List of extracted phone numbers.
"""
phone_numbers = []
pattern = r'\d{3}-\d{3}-\d{4}'
for match in re.findall(pattern, html):
phone_numbers.append(match)
return phone_numbers
def extract_email_addresses(html):
"""
Extracts email addresses from HTML content.
Args:
html: Webpage HTML content.
Returns:
List of extracted email addresses.
"""
email_addresses = []
pattern = r'[a-zA-Z0-9._%+-]+@[a-zA-Z0-9.-]+\.[a-zA-Z]{2,}'
for match in re.findall(pattern, html):
email_addresses.append(match)
return email_addresses
```
**Code Logic Analysis:**
* The `extract_phone_numbers` function uses a regular expression to extract phone numbers from HTML content.
* The `extract_email_addresses` function uses a regular expression to extract email address
0
0
相关推荐
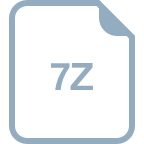
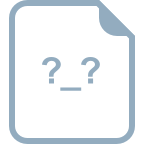
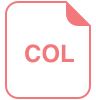
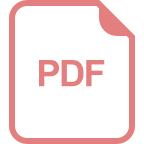
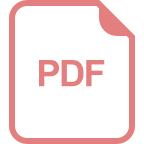
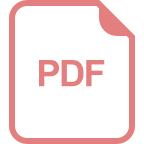
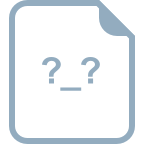
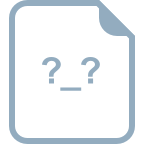