【Advanced Chapter】Advanced Web Crawler Data Storage and Management Strategies: Storing Crawler Data with Elasticsearch
发布时间: 2024-09-15 12:55:22 阅读量: 26 订阅数: 32 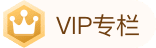
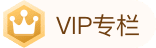
# Advanced篇: Strategies for Data Storage and Management in Advanced Web Scrapers Using Elasticsearch
## 2.1 Fundamental Concepts and Architecture of Elasticsearch
Elasticsearch is a distributed, scalable search and analytics engine built on top of Apache Lucene. It offers a RESTful API that allows users to index, search, and analyze data.
The architecture of Elasticsearch consists of the following components:
- **Nodes:** Single server instances within an Elasticsearch cluster.
- **Cluster:** A collection of multiple nodes that form an Elasticsearch instance.
- **Index:** A logical container for storing a collection of documents.
- **Documents:** Individual data units stored in an index.
- **Fields:** Key-value pairs within a document that describe specific attributes.
- **Shards:** Horizontal partitions of an index, distributed across the nodes in a cluster.
- **Replicas:** Redundant copies of shards for fault tolerance and improved availability.
## 2. Introduction to Elasticsearch and Its Principles
### 2.1 Fundamental Concepts and Architecture of Elasticsearch
Elasticsearch is a distributed, scalable search and analytics engine built on top of Apache Lucene, offering a robust platform for storing, searching, and analyzing vast amounts of data.
Elasticsearch employs a master-slave architecture, comprising the following components:
- **Nodes:** Each server in the Elasticsearch cluster is known as a node. Nodes can be master nodes or data nodes.
- **Master Node:** Responsible for managing and coordinating the cluster, including index allocation, shard routing, and failover.
- **Data Nodes:** Responsible for storing and processing data, including indexing, searching, and aggregation operations.
- **Shards:** Indices are divided into smaller units called shards, allowing for scalability and high availability.
- **Replicas:** Each shard has one or more replicas to enhance data redundancy and availability.
### 2.2 Indexing, Documents, and Fields in Elasticsearch
**Index:** An index is a logical container for storing data in Elasticsearch. It contains one or more documents and defines the structure and fields of the documents.
**Document:** A document is a single data item stored in Elasticsearch. It consists of a set of fields, each with a name and a value.
**Field:** Fields are the data units within a document. They can be of various types, including text, numbers, dates, and booleans. The type of a field determines how Elasticsearch stores, indexes, and searches the data.
### 2.3 Querying and Aggregations in Elasticsearch
Elasticsearch provides a rich set of querying and aggregation features for retrieving and analyzing data from indices.
**Querying:** Queries are used to retrieve documents from an index. They can be based on field values, ranges, or other conditions.
**Aggregation:** Aggregations are used to summarize and group data within an index. They can compute statistics such as sum, average, and count.
Elasticsearch uses a language called Query DSL (Domain-Specific Language) to define queries and aggregations. DSL offers powerful expression capabilities, allowing users to create complex and efficient queries.
**Code Block:**
```
GET /my-index/_search
{
"query": {
"match": {
"title": "Elasticsearch"
}
}
}
```
**Logical Analysis:**
This query uses a match query to search for documents in the my-index index where the value of the title field is "Elasticsearch".
**Parameter Explanation:**
- GET: HTTP request method
- /my-index/_search: Elasticsearch search endpoint
- query: Query object
- match: Type of match query
- title: Field name to match
- Elasticsearch: Value of the field to match
## 3. Using Elasticsearch to Store Web Scraper Data
### 3.1 Modeling and Indexing Web Scraper Data
Before storing web scraper data in Elasticsearch, data modeling and indexing are required. Modeling refers to defining the data structure and field types, while indexing involves creating a data structure to improve query efficiency.
**Modeling**
Data in Elasticsearch is stored in the form of documents, which are composed of fields. Fields can be of various types, such as strings, numbers, dates, etc. When modeling, consider the following factors:
***Data Types:** Choose appropriate field t
0
0
相关推荐
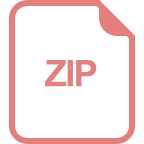
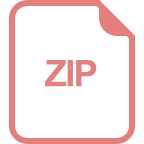
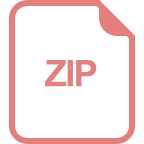
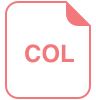
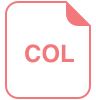
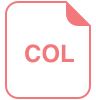
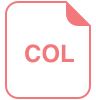
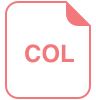
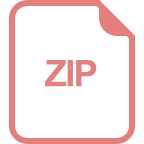